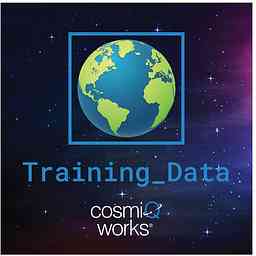
Season 1 Sign Off
1m
·
Training_Data
·
Thank you to everyone who made the inaugural season of Training_Data possible! We will be back with new content for Season 2 in January 2020. Have a great holiday season and happy new year.
The episode Season 1 Sign Off from the podcast Training_Data has a duration of
1:20. It was first published
More episodes from Training_Data
#29 Celebrating CosmiQ Works
Founded in 2015 as part of IQT Labs, CosmiQ Works launched to focus on the geospatial analytics market and provide technical insights, targeted research, reports, and more. Over the past six years CosmiQ has produced many projects and insights that have helped the intelligence and academic communities better understand how geospatial can help tackle hard problems. Our final Training_Data podcast brings together CosmiQ's current colleagues and alum in a special episode celebrating the team's key accomplishments, milestones, and lessons learned through the years.
#28 Introducing the SpaceNet 7 Challenge: Multi-Temporal Urban Development
Satellite imagery analytics have numerous human development and disaster response applications, particularly when time series methods are involved. The Multi-Temporal Urban Development SpaceNet 7 Challenge focuses on developing novel computer vision methods for non-video time series data, asking participants to identify and track buildings in satellite imagery time series collected over rapidly urbanizing areas. In this episode, CosmiQ’s Ryan Lewis, Adam Van Etten, and Daniel Hogan are joined by Planet’s Jesus Martinez Manzo and AWS Disaster Response’s Grace Kitzmiller to explore this new challenge.
Learn more at www.spacenet.ai, and at the DownLinQ (https://medium.com/the-downlinq)
#27 SpaceNet 6: The Multi-Modal Extravaganza Family Hour
CosmiQ’s Jake Shermeyer and Daniel Hogan are joined by Capella Space’s Jason Brown and IEEE Geoscience and Remote Sensing’s (GRSS) Ronny Hänsch to once again discuss the SpaceNet 6 Dataset and post-challenge experiments. Learn more about data fusion and deep learning approaches that work to blend synthetic aperture radar (SAR) and optical imagery. Additionally, the podcast also explores the value of frequent SAR revisits that can be beneficial for foundational mapping applications. Finally, the group discusses CosmiQ’s new addition to the Solaris python package: a multi-modal pre-processing library and a new API called ‘PipeSegment’ for seamlessly stringing together different operations.
SpaceNet is a nonprofit made possible by co-founder and managing partner, CosmiQ Works; co-founder and co-chair, Maxar Technologies; and all the other Partners: Amazon Web Services (AWS), Capella Space, Topcoder, IEEE Geoscience and Remote Sensing (GRSS), the National Geospatial-Intelligence Agency, and Planet.
#26 SpaceNet 6 Challenge Results: Multi-Sensor All-Weather Mapping
SpaceNet is a non-profit dedicated to accelerating open source, applied research in geospatial machine learning. In this episode, CosmiQ’s Ryan Lewis, Jake Shermeyer, and Daniel Hogan discuss the SpaceNet 6 Challenge where participants were asked to automatically extract building footprints with computer vision and AI algorithms using a combination of synthetic aperture radar (SAR) and electro-optical imagery. Hear about the challenge’s winning artificial intelligence models and the tradeoff between inference speed and model performance.
SpaceNet is made possible by co-founder and managing partner, CosmiQ Works; co-founder and co-chair, Maxar Technologies; and all the other Partners: Amazon Web Services (AWS), Capella Space, Topcoder, IEEE Geoscience and Remote Sensing (GRSS), the National Geospatial-Intelligence Agency, and Planet.
Learn more at www.spacenet.ai, and at the DownLinQ (https://medium.com/the-downlinq)
#25 Evaluating SpaceNet 5 Challenge Results: Road Network Detection & Optimized Routing
Despite its application to myriad humanitarian and civil use cases, automated road network extraction from overhead satellite imagery remains quite challenging. However, the SpaceNet 5 challenge made significant progress in this field with top participants being able to extract both road networks and speed/travel time estimates for each roadway. On today’s pod, CosmiQ’s Ryan Lewis and Dr. Adam Van Etten explore the challenge’s unique dataset and geographic diversity over time, the winning models, and the tradeoff between inference speed and model performance.
SpaceNet is a non-profit LLC co-founded and managed by In-Q-Tel's CosmiQ Works in collaboration Maxar Technologies, a co-founder, and the other SpaceNet Partners including AWS, Intel AI, Topcoder, Capella Space, IEEE GRSS, The National Geospatial-Intelligence Agency (NGA), and Planet.