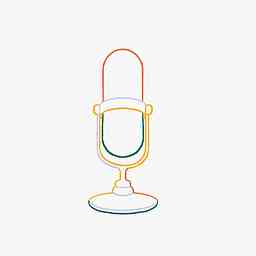
Ground Truths
by Eric Topol
Facts, data, and analytics about biomedical matters.
erictopol.substack.com
Copyright: Eric Topol
Episodes
Akiko Iwasaki: The Immunology of Covid and the Future
41m · PublishedIf there’s one person you’d want to talk to about immunology, the immune system and Covid, holes in our knowledge base about the complex immune system, and where the field is headed, it would be Professor Iwasaki. And add to that the topic of Women in Science. Here’s our wide-ranging conversation.
A snippet of the video, Full length Ground Truths videos are posted here and you can subscribe.
Ground Truths is a reader-supported publication. To receive new posts and support my work, consider becoming a free or paid subscriber.
Transcript with many external link and links to the audio, recorded 30 April 2024
Eric Topol (00:06):
Hello, it's Eric Topol and I'm really thrilled to have my friend Akiko Iwasaki from Yale, and before I start talking with Akiko, I just want to mention there aren't too many silver linings of the pandemic, but one for me was getting to know Professor Iwasaki. She is my go-to immunologist. I've learned so much from her over the last four years and she's amazing. She just, as you may know, she was just recently named one of the most influential people in the world by TIME100. [and also recognized this week in TIME 100 Health]. And besides that, she's been elected to the National Academy of Medicine, National Academy of Sciences. She's the president of the American Association of Immunologists and she's a Howard Hughes principal investigator. So Akiko, it's wonderful to have you to join into an extended discussion of things that we have of mutual interest.
Akiko Iwasaki (01:04):
Thank you so much, Eric, for having me. I equally appreciate all of what you do, and I follow your blog and tweets and everything. So thank you Eric.
Eric Topol (01:14):
Well, you are a phenom. I mean just, that's all I can say because I think it was so appropriate that TIME recognize your contributions, not just over the pandemic, but of course throughout your career, a brilliant career in immunology. I thought we'd start out with our topic of great interest on Long Covid. You've done seminal work here and this is an evolving topic obviously. I wonder what your latest thoughts are on the pathogenesis and where things are headed.
Long Covid
Akiko Iwasaki (01:55):
Yeah, so as I have been saying throughout the pandemic, I think that Long Covid is not one disease. It's a collection of multiple diseases and that are sort of ending up in similar sets of symptoms. Obviously, there are over 200 symptoms and not everyone has the same set of symptoms, but what we are going for is trying to understand the disease drivers, so persistent viral infection is one of them. There are overwhelming evidence for that theory now, all the way from autopsy and biopsy studies to looking at peripheral blood RNA signatures as well as circulating spike protein and nucleocapsid proteins that are detected in people with Long Covid. Now whether that persistent virus or remnants of virus is driving the disease itself is unclear still. And that's why trials like the one that we are engaging with Harlan Krumholz on Paxlovid should tell us what percentage of the people are suffering from that type of driver and whether antivirals like Paxlovid might be able to mitigate those. If I may, I'd like to talk about three other hypotheses.
Eric Topol (03:15):
Yeah, I'd love for you to do that.
Akiko Iwasaki (03:18):
Okay, great. So the second hypothesis that we've been working on is autoimmune disease. And so, this is clearly happening in a subset of people, again, it's a heterogeneous disease, but we can actually not only look at reactogenicity of antibodies from people with Long Covid where we can transfer IgG from patients with Long Covid into an animal, a healthy animal, and really measure outcomes of a pathogenesis. So that's a functional evidence that antibodies in some people with Long Covid is really actually causing some of the damages that are occurring in vivo. And the third hypothesis is the reactivation of herpes viruses. So many of us adults have multiple latent herpes virus family members that are just dormant and are not really causing any pathologies. But in people with Long Covid, we're seeing elevated reactivation of viruses like Epstein-Barr virus (EBV) or Varicella-zoster virus (VZV) and that may again be just a signature of Long Covid, but it may also be driving some of the symptoms that people are suffering from.
(04:32):
So that's again, we see the signature over and over, not just our group, but multiple other groups, Michael Peluso's group, Jim Heath, and many others. So that's also an emerging evidence from multiple groups showing that. And finally, we think that inflammation that occurs during the acute phase can sort of chronically change some tissue tone. For instance, in the brain with Michelle Monje’s team, we developed a sort of localized mild Covid model of infection and showed that changes in microglia can be seen seven weeks post infection even though the virus is completely gone. So that means that inflammation that's established as a result of this initial infection can have prolonged sequence and sequela within the person and that may also be driving disease. And Eric, the reason we need to understand these diseases separately is because not only for diagnostic purposes, but for therapeutic purposes because to target a persistent virus is very different approach from targeting autoantibodies, for example.
Eric Topol (05:49):
Well, that's great. There's a lot to unpack there as you laid out four distinct paths that could result in the clinical syndrome and sequelae. I think you know I had the chance to have a really fun conversation with Michelle about their joint work that you've done, and she reminded me how she made a cold call to you to start as a collaboration, which I thought was fantastic. Look what that yielded. But yeah, this is fascinating because as I think you're getting at is that it may not be the same pathogenesis in any given individual so that all these, and even others might be operative. I guess maybe I first delve into the antibody story as you're well aware, we see after people get Covid a higher rate of autoimmune diseases crop up, which is really interesting because it seems to rev up self-directed immune response. And this I think many people haven't really noted yet, although obviously you're well aware of this, it's across all the different autoimmune diseases, connective tissue disease, not just one in particular. And it's, as you say, the idea that you could take the blood from a person suffering from Long Covid and give it to an experimental animal model and be able to recapitulate some of the abnormalities, it's really pretty striking. So the question I guess is if you were to do plasmapheresis and try to basically expunge these autoantibodies, wouldn't you expect people to have some symptomatic benefit pretty rapidly or is it just that the process is already far from the initiating step?
Akiko Iwasaki (07:54):
That's a great question. Plasmapheresis may be able to transiently improve the person if they're suffering from these autoantibody mediated diseases. People have reported, for example, IVIG treatment has dramatically improved their symptoms, but not in everybody. So it's really critical to understand who's suffering from this particular driver and appropriately treat those people. And there are many other very effective therapies in autoimmune disease field that can be repurposed for treating these patients as well.
Eric Topol (08:34):
The only clinical trial that has clicked so far, interestingly, came out of Hong Kong with different types of ways to manipulate the gut microbiome, which again, you know better than me is a major modulator of our immune system response. What are your thoughts about taking advantage of that way to somehow modulate this untoward immune response in people with this condition?
Akiko Iwasaki (09:07):
Yeah, so that is an exciting sort of development, and I don't mean to discount the importance of microbiome at all. It's just the drivers that are mentioning are something that can be directly linked to disease, but certainly dysbiosis and translocation of metabolites and microbiome itself could trigger Long Covid as well. So it's something that we're definitely keeping our eyes on. And as you say, Eric, the immune system is in intimate contact with the gut microbiome and also the gut is intimate contact with the brain. So there's a lot of connections that we really need to be paying attention to. So yeah, absolutely. This is a very exciting development.
Eric Topol (09:57):
And it is intriguing of course, the reactivation of viruses. I mean, we’ve learned in recent years how important EBV is in multiple sclerosis (MS). The question I have for you on that pathway, is this just an epiphenomena or do you actually think that could be a driving force in some people?
Akiko Iwasaki (10:19):
Yeah, so that's really hard to untangle in people. I mean, David Putrino and my team we're planning a clinical trial using Truvada. Truvada obviously is an HIV drug, but it has reported antiviral activity to Epstein-Barr virus (EBV) and others. So potentially we can try to interrogate that in people, but we're also developing mouse models that can sort of recapitulate EBV like viral reactivation and to see whether there's any sort of causal link between the reactivation and disease process.
Eric Topol (10:57):
Right now, recently there's been a bunch of anecdotes of people who get the glucagon-like peptide one (GLP-1) drugs which have a potent anti-inflammatory, both systemic and in the brain. I'd love to test these drugs, but of course these companies that make them or have other interests outside of Long Covid, do you think there's potential for a drug lik
Aviv Regev: The Revolution in Digital Biology
36m · Published“Where do I think the next amazing revolution is going to come? … There’s no question that digital biology is going to be it. For the very first time in our history, in human history, biology has the opportunity to be engineering, not science.”—Jensen Huang, NVIDIA CEO
Aviv Regev is one of the leading life scientists of our time. In this conversation, we cover the ongoing revolution in digital biology that has been enabled by new deep knowledge on cells, proteins and genes, and the use of generative A.I .
Transcript with audio and external links
Eric Topol (00:05):
Hello, it's Eric Topol with Ground Truths and with me today I've really got the pleasure of welcoming Aviv Regev, who is the Executive Vice President of Research and Early Development at Genentech, having been 14 years a leader at the Broad Institute and who I view as one of the leading life scientists in the world. So Aviv, thanks so much for joining.
Aviv Regev (00:33):
Thank you for having me and for the very kind introduction.
The Human Cell Atlas
Eric Topol (00:36):
Well, it is no question in my view that is the truth and I wanted to have a chance to visit a few of the principal areas that you have been nurturing over many years. First of all, the Human Cell Atlas (HCA), the 37 trillion cells in our body approximately a little affected by size and gender and whatnot, but you founded the human cell atlas and maybe you can give us a little background on what you were thinking forward thinking of course when you and your colleagues initiated that big, big project.
Aviv Regev (01:18):
Thanks. Co-founded together with my very good friend and colleague, Sarah Teichmann, who was at the Sanger and just moved to Cambridge. I think our community at the time, which was still small at the time, really had the vision that has been playing out in the last several years, which is a huge gratification that if we had a systematic map of the cells of the body, we would be able both to understand biology better as well as to provide insight that would be meaningful in trying to diagnose and to treat disease. The basic idea behind that was that cells are the basic unit of life. They're often the first level at which you understand disease as well as in which you understand health and that in the human body, given the very large number of individual cells, 37.2 trillion give or take, and there are many different characteristics.
(02:16):
Even though biologists have been spending decades and centuries trying to characterize cells, they still had a haphazard view of them and that the advancing technology at the time – it was mostly single cell genomics, it was the beginnings also of spatial genomics – suggested that now there would be a systematic way, like a shared way of doing it across all cells in the human body rather than in ways that were niche and bespoke and as a result didn't unify together. I will also say, and if you go back to our old white paper, you will see some of it that we had this feeling because many of us were computational scientists by training, including both myself and Sarah Teichmann, that having a map like this, an atlas as we call it, a data set of this magnitude and scale, would really allow us to build a model to understand cells. Today, we call them foundational models or foundation models. We knew that machine learning is hungry for these kinds of data and that once you give it to machine learning, you get amazing things in return. We didn't know exactly what those things would be, and that has been playing out in front of our eyes as well in the last couple of years.
Spatial Omics
Eric Topol (03:30):
Well, that gets us to the topic you touched on the second area I wanted to get into, which is extraordinary, which is the spatial omics, which is related to the ability to the single cell sequencing of cells and nuclei and not just RNA and DNA and methylation and chromatin. I mean, this is incredible that you can track the evolution of cancer, that the old word that we would say is a tumor is heterogeneous, is obsolete because you can map every cell. I mean, this is just changing insights about so much of disease health mechanisms, so this is one of the hottest areas of all of life science. It's an outgrowth of knowing about cells. How do you summarize this whole era of spatial omics?
Aviv Regev (04:26):
Yeah, so there's a beautiful sentence in the search for lost time from Marcel Proust that I'm going to mess up in paraphrasing, but it is roughly that going on new journeys is not about actually going somewhere physically but looking with new eyes and I butchered the quote completely.[See below for actual quote.] I think that is actually what single cells and then spatial genomics or spatial omics more broadly has given us. It's the ability to look at the same phenomenon that we looked at all along, be it cancer or animal development or homeostasis in the lung or the way our brain works, but having new eyes in looking and because these new eyes are not just seeing more of something we've seen before, but actually seeing things that we couldn't realize were there before. It starts with finding cells we didn't know existed, but it's also the processes that these cells undergo, the mechanisms that actually control that, the causal mechanisms that control that, and especially in the case of spatial genomics, the ways in which cells come together.
(05:43):
And so we often like to think about the cell because it's the unit of life, but in a multicellular organism we just as much have to think about tissues and after that organs and systems and so on. In a tissue, you have this amazing orchestration of the interactions between different kinds of cells, and this happens in space and in time and as we're able to look at this in biology often structure is tightly associated to function. So the structure of the protein to the function of the protein in the same way, the way in which things are structured in tissue, which cells are next to each other, what molecules are they expressing, how are they physically interacting, really tells us how they conduct the business of the tissue. When the tissue functions well, it is this multicellular circuit that performs this amazing thing known as homeostasis.
(06:36):
Everything changes and yet the tissue stays the same and functions, and in disease, of course, when these connections break, they're not done in the right way you end up with pathology, which is of course something that even historically we have always looked at in the level of the tissue. So now we can see it in a much better way, and as we see it in a better way, we resolve better things. Yes, we can understand better the mechanisms that underlie the resistance to therapeutics. We can follow a temporal process like cancer as it unfortunately evolves. We can understand how autoimmune disease plays out with many cells that are actually bent out of shape in their interactions. We can also follow magnificent things like how we start from a single cell, the fertilized egg, and we become 37.2 trillion cell marvel. These are all things that this ability to look in a different way allows us to do.
Eric Topol (07:34):
It's just extraordinary. I wrote at Ground Truths about this. I gave all the examples at that time, and now there's about 50 more in the cardiovascular arena, knowing with single cell of the pineal gland that the explanation of why people with heart failure have sleep disturbances. I mean that's just one of the things of so many now these new insights it's really just so remarkable. Now we get to the current revolution, and I wanted to read to you a quote that I have.
Digital Biology
Aviv Regev (08:16):
I should have prepared mine. I did it off the top of my head.
Eric Topol (08:20):
It's actually from Jensen Huang at NVIDIA about the digital biology [at top of the transcript] and how it changes the world and how you're changing the world with AI and lab in the loop and all these things going on in three years that you've been at Genentech. So maybe you can tell us about this revolution of AI and how you're embracing it to have AI get into positive feedbacks as to what experiment to do next from all the data that is generated.
Aviv Regev (08:55):
Yeah, so Jensen and NVIDIA are actually great partners for us in Genentech, so it's fun to contemplate any quote that comes from there. I'll actually say this has been in the making since the early 2010s. 2012 I like to reflect on because I think it was a remarkable year for what we're seeing right now in biology, specifically in biology and medicine. In 2012, we had the beginnings of really robust protocols for single cell genomics, the first generation of those, we had CRISPR happen as a method to actually edit cells, so we had the ability to manipulate systems at a much better way than we had before, and deep learning happened in the same year as well. Wasn't that a nice year? But sometimes people only realize the magnitude of the year that happened years later. I think the deep learning impact people realized first, then the single cells, and then the CRISPR, then the single cells.
(09:49):
So in order maybe a little bit, but now we're really living through what that promise can deliver for us. It's still the early days of that, of the delivery, but we are really seeing it. The thing to realize there is that for many, many of the problems that we try to solve in biomedicine, the problem is bigger than we would ever be able to perform experiments or collect data. Even if we had the genomes of all the people in the world, all billions and billions of them, that's just a smidge compared to all of the ways in which their common var
Jennifer Doudna: The Exciting Future of Genome Editing
31m · PublishedProfessor Doudna was awarded the 2020 Nobel Prize in Chemistry with Professor Emmanuelle Charpentier for their pioneering work in CRISPR genome editing. The first genome editing therapy (Casgevy) was just FDA approved, only a decade after the CRISPR-Cas9 editing system discovery. But It’s just the beginning of a much bigger impact story for medicine and life science.
Ground Truths podcasts are now on Apple and Spotify.
And if you prefer videos, they are posted on YouTube
Transcript with links to audio and relevant external links
Eric Topol (00:06):
This is Eric Topol with Ground Truths, and I'm really excited today to have with me Professor Jennifer Doudna, who heads up the Innovative Genomics Institute (IGI) at UC Berkeley, along with other academic appointments, and as everybody knows, was the Nobel laureate for her extraordinary discovery efforts with CRISPR genome editing. So welcome, Jennifer.
Jennifer Doudna (00:31):
Hello, Eric. Great to be here.
Eric Topol (00:34):
Well, you know we hadn't met before, but I felt like I know you so well because this is one of my favorite books, The Code Breaker. And Walter Isaacson did such a wonderful job to tell your story. What did you think of the book?
My interview with Walter Isaacson on The Code Breaker, a book I highly recommend
Jennifer Doudna (00:48):
I thought Walter did a great job. He's a good storyteller, and as you know from probably from reading it or maybe talking to others about it, he wrote a page turner. He actually really dug into the science and all the different aspects of it that I think created a great tale.
Eric Topol (01:07):
Yeah, I recommended highly. It was my favorite book when it came out a couple years ago, and it is a page turner. In fact, I just want to read one, there's so many quotes out of it, but in the early part of the book, he says, “the invention of CRISPR and the plague of Covid will hasten our transition to the third great revolution of modern times. These revolutions arose from the discovery beginning just over a century ago, of the three fundamental kernels of our existence, the atom, the bit, and the gene.” That kind of tells a big story just in one sentence, but I thought I’d start with the IGI, the institute that you have set up at Berkeley and what its overall goals are.
Jennifer Doudna (01:58):
Right. Well, let's just go back a few years maybe to the origins of this institute and my thinking around it, because in the early days of CRISPR, it was clear that we were really at a moment that was quite unique in the sense that there was a transformative technology. It was going to intersect with lots of other discoveries and technologies. And I work at a public institution and my question to myself was, how can I make sure that this powerful tool is first of all used responsibly and secondly, that it's used in a way that benefits as many people as possible, and it's a tall order, but clearly we needed to have some kind of a structure that would allow people to work together towards those goals. And that was really the mission behind the IGI, which was started as a partnership between UC Berkeley and UCSF and now actually includes UC Davis as well.
The First FDA Approved Genome Editing
Eric Topol (02:57):
I didn't realize that. That's terrific. Well, this is a pretty big time because 10 years or so, I guess starting to be 11 when you got this thing going, now we're starting to see, well, hundreds of patients have been treated and in December the FDA approved the first CRISPR therapy for sickle cell disease, Casgevy. Is that the way you say it?
Jennifer Doudna (03:23):
Casgevy, yeah.
Eric Topol (03:24):
That must have felt pretty good to see if you go from the molecules to the bench all the way now to actually treating diseases and getting approval, which is no easy task.
Jennifer Doudna (03:39):
Well, Eric, for me, I'm a biochemist and somebody who has always worked on the fundamentals of biology, and so it's really been extraordinary to see the pace at which the CRISPR technology has been adopted, and not just for fundamental research, but also for real applications. And Casgevy is sort of the crowning example of that so far, is that it's really a technology that we can already see how it's being used to, I think it's fair to say, effectively cure a genetic disease for the first time. Really amazing.
Genome Editing is Not the Same as Gene Therapy
Eric Topol (04:17):
Yeah. Now I want to get back to that. I know there's going to be refinements about that. And of course, there's beta thalassemia, so we've got two already, and our mutual friend Fyodor Urnov would say two down 5,000 to go. But I think before I get to the actual repair of the sickle cell defect molecular defect, I think one of the questions I think that people listeners may not know is the differentiation of genome editing with gene therapy. I mean, as you know, there was recently a gene therapy approval for something like $4.25 million for metachromatic leukodystrophy. So maybe you could give us kind of skinny on how these two fundamental therapies are different.
Jennifer Doudna (05:07):
Right. Well, it's a great question because the terminology sounds kind of the same, and so it could be confusing. Gene therapy goes back decades, I can remember gene therapy being discussed as an exciting new at the time, direction back when I was a graduate student. That was little while ago. And it refers to the idea that we can use a genetic approach for disease treatment or even for a cure. However, it fundamentally requires some mechanism of integrating new information into a genome. And traditionally that's been done using viruses, which are great at doing that. It's just that they do it wherever they want to do it, not necessarily where we want that information to go. And this is where CRISPR comes in. It's a technology allows precision in that kind of genetic manipulation. So it allows the scientist or the clinician to decide where to make a genetic change. And that gives us tremendous opportunity to do things with a kind of accuracy that hasn't been possible before.
Eric Topol (06:12):
Yeah, no question. That's just a footnote. My thesis in college at University of Virginia, 1975, I'm an old dog, was prospects for gene therapy in man. So it took a while, didn't it? But it's a lot better now with what you've been working on, you and your colleagues now and for the last decade for sure. Now, what I was really surprised about is it's not just of course, these hemoglobin disorders, but now already in phase two trials, you've got hereditary angioedema, which is a life-threatening condition, amyloidosis, cancer ex vivo, and also chronic urinary tract infections. And of course, there's six more others like autoimmune diseases like lupus and type 1 diabetes. So this is really blossoming. It's really extraordinary.
Eric Topol (07:11):
I mean, wow. So one of the questions I had about phages, because this is kind of going back to this original work and discovery, antimicrobial resistance is really a big problem and it's a global health crisis, and there's only two routes there coming up with new drugs, which has been slow and not really supported by the life science industry. And the other promising area is with phages. And I wonder, since this is an area you know so well, why haven't we put more, we're starting to see more trials in phages. Why haven't we doubled down or tripled down on this to help the antimicrobial resistance problem?
Jennifer Doudna (08:00):
Well, it's a really interesting area, and as you said, it's kind of one of those areas of science where I think there was interest a while ago and some effort was made for reasons that are not entirely clear to me, at least it fizzled out as a real focused field for a long time. But then more recently, people have realized that there's an opportunity here to take advantage of some natural biology in which viruses can infect and destroy microbes. Why aren't we taking better advantage of that for our own health purposes? So I personally am very excited about this area. I think there's a lot of fundamental work still to be done, but I think there's a tremendous opportunity there as well.
CRISPR 2.0
Eric Topol (08:48):
Yeah, I sure think we need to invest in that. Now, getting back to this sickle cell story, which is so extraordinary. This is kind of a workaround plan of getting fetal hemoglobin built up, but what about actually repairing, getting to fixing the lesion, if you will?
Eric Topol (09:11):
Yeah. Is that needed?
Jennifer Doudna (09:13):
Well, maybe it's worth saying a little bit about how Casgevy works, and you alluded to this. It's not a direct cure. It's a mechanism that allows activation of a second protein called fetal hemoglobin that can suppress the effect of the sickle cell mutation. And it's great, and I think for patients, it offers a really interesting opportunity with their disease that hasn't been available in the past, but at the same time, it's not a true cure. And so the question is could we use a CRISPR type technology to actually make a correction to the genetic defect that directly causes the disease? And I think the answer is yes. The field isn't there quite yet. It's still relatively difficult to control the exact way that DNA editing is occurring, especially if we're doing it in vivo in the body. But boy, many people are working on this, as you probably know. And I really think that's on the horizon.
Eric Topol (10:19):
Yeah. Well, I think we want to get into the in vivo story as well because that, I think right now it's so complica
Daniel Drucker: Illuminating the GLP-1 Drug's Break Out
36m · PublishedNote: This podcast is a companion to the Ground Truths newsletter “A Big Week for GLP-1 Drugs”
Eric Topol (00:06):
It is Eric Topol with Ground Truths, and with me today is Dr. Daniel Drucker from the University of Toronto, who is one of the leading endocrinologists in the world, and he along with Joel Habener and Jens Juul Holst from the University of Copenhagen and Denmark, have been credited with numerous prizes of their discovery work of glucagon-like peptide-1 (GLP-1) as we get to know these family of drugs and he's a true pioneer. He's been working on this for decades. So welcome, Daniel.
Daniel Drucker (00:43):
Thank you.
Eric Topol (00:45):
Yeah, it's great to have you and to get the perspective, one of the true pioneers in this field, because to say it's blossom would be an understatement, don't you think?
Daniel Drucker (00:57):
Yeah, it's been a bit of a hectic three years. We had a good quiet 30 plus years of solid science and then it's just exploded over the last few years.
Eric Topol (01:06):
Yeah, back in 30 years ago, did you have any sense that this was coming?
Daniel Drucker (01:14):
Not what we're experiencing today, I think there was a vision for the diabetes story. The first experiments were demonstrating insulin secretion and patents were followed around the use for the treatment of GLP-1 for diabetes. The food intake story was much more gradual and the weight loss story was quite slow. And in fact, as you know, we've had a GLP-1 drug approved for people with obesity since 2014, so it's 10 years since liraglutide was approved, but it didn't really catch the public's attention. The weight loss was good, but it wasn't as spectacular as what we're seeing today. So this really has taken off just over the last three, four years.
Eric Topol (01:58):
Yeah, no, it's actually, I've never seen a drug class like this in my life, Daniel. I mean, I've obviously witnessed the statins, but this one in terms of pleiotropy of having diverse effects, and I want to get to the brain here in just a minute because that seems to be quite a big factor. But one thing just before we get too deep into this, I think you have been great to recognize one of your colleagues who you work with at Harvard, Svetlana Mojsov. And the question I guess is over the years, as you said, there was a real kind of incremental path and I guess was in 1996 when you said, well, this drug likely will inhibit food intake, but then there were gaps of many years since then, as you mentioned about getting into the obesity side. Was that because there wasn't much weight loss in the people with diabetes or was it related to the dose of the drugs that were being tested?
Why Did It Take So Long to Get to Obesity?
Daniel Drucker (03:11):
Well, really both. So the initial doses we tested for type 2 diabetes did not produce a lot of weight loss, maybe 2-3%. And then when we got semaglutide for type 2 diabetes, maybe we were getting 4-5% mean weight loss. And so that was really good and that was much better than we achieved before with any glucose lowering drug. But a lot of credit goes to Novo Nordisk because they looked at the dose for liraglutide and diabetes, which was 1.8 milligrams once daily for people with type 2 diabetes. And they asked a simple question, what if we increase the dose for weight loss? And the answer was, we get better weight loss with 3 milligrams once a day. So they learn that. And when they introduced semaglutide for type 2 diabetes, the doses were 0.5 and 1 milligrams. But in the back of their minds was the same question, what if we increased the dose and they landed on 2.4 milligrams once a week. And that's when we really started to see that the unexpected spectacular weight loss that we're now quite familiar with.
Eric Topol (04:16):
Was there also something too that diabetics don't lose as much weight if you were to have match dose?
Daniel Drucker (04:22):
Yeah, that's a general phenomenon. If one goes from either diet to bariatric surgery, and certainly with weight loss medicines, we tend to see maybe two thirds to three quarters of the amount of weight loss in people with type 2 diabetes. We don't really understand it. The brain pathways are probably resistant to some of the pathways that are activated that lead to weight loss, and it's really an interesting observation that needs further study.
The Brain Effect
Eric Topol (04:50):
Yeah, it's fascinating really. And it might've at least in part, held up this progress that has been truly remarkable. Now, recently you published a paper among many, you're a very prolific scientist, of course, physician scientist, but back in December in Cell Metabolism was a very important paper that explored the brain gut axis, the ability to inhibit inflammation and the mechanism through Toll-like receptors that you were seeing that. So maybe you could summarize the fact that you saw this, you were quoted in this Atlantic piece by Sarah Zhang, the science behind Ozempic was wrong. The weight loss effects of GLP-1 drugs have little to do with the gut and basically claiming that it's related to the effects on the brain, which of course could be reduced inflammation, reduced or inhibiting centers of addiction craving, that sort of thing. So how do you interpret your recent results and ongoing studies regarding GLP-1's effect on the brain?
Daniel Drucker (06:02):
Sure, so to be clear, I don't think that was a quote. I never would've said the science behind Ozempic was wrong. I think that was a headline writer doing what they do best, which is catching people's attention. I think what I was trying to say is that where this field started with insulin secretion first and then weight loss second, those are clearly very important pharmacological attributes of GLP-1. But physiologically, if we take GLP-1 away or we take the receptor away, you don't really develop diabetes without GLP-1. You don't really gain a lot of weight without GLP-1. So physiologically it's not that important. Why do we have GLP-1 in the distal gut? I think physiologically it's there to defend against infection and reduce gut inflammation. But we noticed that GLP-1 reduces inflammation in many different places in the heart and blood vessels and in the liver and many organs where you don't see a lot of GLP-1 receptors and you don't see a lot of GLP-1 receptors on immune cells.
Daniel Drucker (07:04):
So that really led us to the question, well, how does it work and affect all these organs where we don't see a lot of the receptors? And that's where we landed on the brain. Obviously the nervous system can communicate with many different cell types in almost every organ. And we identified neurons that expressed the GLP-1 receptor, which when blocked abrogated or completely eliminated the ability of GLP-1 to reduce inflammation in the periphery in white cells or in lungs. So it's been known for some time that the brain can control the immune system. So this is just the latest piece in the puzzle of how GLP-1 might reduce inflammation.
Eric Topol (07:49):
And just to be clear, I was quoting the Atlantic headline, not you that you were quoted within that article, but this is something that's really interesting because obviously GLP-1 is made in the brain in certain parts of the brain, it's transient in terms of its half-life made from the gut. But when we give these drugs, these agonists, how does it get in the brain? Because isn't there a problem with the blood brain barrier?
Daniel Drucker (08:22):
So I don't think the drugs get into the brain very well. We have a lot of data on this, so people have done the classic experiments, they either make radioactive ligands or fluorescent ligands, and they look how much gets in it and not very much gets in beyond the blood-brain barrier. And we also have big drugs that are immunoglobulin based and they work really well, so they don't get into the brain very much at all. And so, the way I describe this is that GLP-1 talks to the brain, but it doesn't directly get into the brain to meaningful extent, it does communicate somewhat there are areas obviously that are accessible in the area of the stream and circumventricular organs, but most of the time we have this communication that's not well understood that results in the magic that we see. And there are some discussions around for the neurodegenerative disease story where GLP-1 is being looked at in Parkinson's disease and in people with Alzheimer's disease. Would you be able to get more benefit if you could get the drugs into the brain to a greater extent, or would you simply increase the adverse event profile and the adverse response? So really important area for study as we begin to go beyond diabetes and obesity.
Eric Topol (09:41):
Yeah, I mean as you're pointing out, there's two ongoing trials, pretty large trials in Alzheimer's, early Alzheimer's, which may be a little bit too late, but at any rate, testing GLP-1 to see whether or not it could help prevent progression of the disease. And as you also mentioned, diseases and Parkinson's. But I guess, so the magic as you referred to it, the gut -brain axis so that when you give the GLP-1 family of drugs, we'll talk more about the double and triple receptor in a moment, but when you give these drugs, how does the message you get from the gut to the brain would you say?
Daniel Drucker (10:27):
So pharmacologically, we can give someone or an animal the drug, it does reach some of the accessible neurons that have GLP-1 receptors, and they probably transmit signals deeper into the brain and then activate signal transduction. So one way to look at it, if you use c-fos, the protein, which is an immediate
Sid Mukherjee: On A.I., Longevity and Being A Digital Human
47m · PublishedSiddhartha Mukherjee is a Professor at Columbia University, oncologist, and extraordinary author of Emperor of All Maladies (which was awarded a Pulitzer Prize), The Gene, and The Song of the Cell, along with outstanding pieces in the New Yorker. He is one of the top thought leaders in medicine of our era.
“I have begun to imagine, think about what it would be to be a digital human..”—Sid Mukherjee
Eric Topol (00:06):
Well, hello, this is Eric Topol with Ground Truths, and I am delighted to have my friend Sid Mukherjee, to have a conversation about all sorts of interesting things. Sid, his most recent book, SONG OF THE CELL is extraordinary. And I understand, Sid, you're working on another book that may be cell related. Is that right?
Sid Mukherjee (00:30):
Eric, it's not cell related, I would say, but it's AI and death related, and it covers, broadly speaking, it covers AI, longevity and death and memory —topics that I think are universal, but also particularly medicine.
Eric Topol (00:57):
Well, good, and we'll get into that. I had somehow someone steered me that your next book was going to be something building on the last one, but that sounds even more interesting. You're going in another direction. You've covered cancer gene cells, so I think covering this new topic is of particularly interest. So let's get into the AI story and maybe we'll start off with your views on the healthcare side. Where do you think this is headed now?
A.I. and Drug Discovery
Sid Mukherjee (01:29):
So I think Eric, there are two very broad ways of dividing where AI can enter healthcare, and there may be more, I'm just going to give you two, but there may be more. One is on what I would call the deep science aspect of it, and by that I mean AI-based drug discovery, AI-based antibody discovery, AI-based modeling. All of which use AI tools but are using tools that have to do with machine learning, but may have to do less directly with the kind of large language models. These tools have been in development for a long time. You and I are familiar with them. They are tools. Very simply put, you can imagine that the docking of a drug to a protein, so imagine every drug, every medicine as a small spaceship that docks onto a large spaceship, the large spaceship being the target.
(02:57):
So if you think of it that way, there are fundamental rules. If anyone's watched Star Wars or any of these sci-fi films, there are fundamental rules by which that govern the way that the small spaceship in this case, a molecule like aspirin fits into a pocket of its target, and those are principles that are determined entirely by chemistry and physics, but they can be taught, you can learn what kind of spaceship or molecule is likely to fit into what kind of pocket of the mothership, in this case, the target. And if they can be learned, they're amenable to AI-based discovery.
Eric Topol (03:57):
Right. Well, that's, isn't that what you'd call the fancy term structure-based discovery, where you're using such tools like what AlphaFold2 for proteins and then eventually for antibodies, small molecules, et cetera, that you can really rev up the whole discovery of new molecules, right?
Sid Mukherjee (04:21):
That's correct, and that's one of the efforts that I'm very heavily involved in. We have created proprietary algorithms that allow us to enable this. Ultimately, of course, there has to be a method by which you start from these AI based methods, then move to physical real chemistry, then move to real biology, then move to obviously human biology and ultimately to human studies. It's a long process, but it's an incredibly fruitful process.
Eric Topol (04:57):
Well, yeah, as an example that recently we had Jim Collins on the podcast and he talked about the first new drug class of antibiotics in two decades that bind to staph aureus methicillin resistant, and now in clinical trials. So it’s happening. There’s 20 AI drugs in clinical trials out there.
Sid Mukherjee (05:18):
It’s bound to happen. It is an unstoppable bound to happen systematology of drug discovery. This is just bound to happen. It is unstoppable. There are kinks in it in the road, but those will be ironed out, but it’s bound to happen.
(05:41):
So that’s on the very discovery oriented end, which is more related to learning algorithms that have to do with AI and less to do with what we see in day-to-day life, the ChatGPT kind of day-to-day life of the world. On the very other end of the spectrum, just to move along on the very other end of the spectrum are what I would call patient informatics. So by patient informatics, I mean questions like who responds to a particular drug? What genes do they have? What environment are they in? Have they had other drug interactions in the past? What is it about their medical record that will allow us to understand better why or why they're not responding to a medicine?
(06:51):
Those are also AI, can also be really powered by AI, but are much more dependent and much more sensitive to our understanding of these current models, the large language models. So just to give you an example, let's say you wanted to enroll a clinical trial for patients with diabetes to take a new drug. You could go into the electronic medical record, which right now is a text file, and ask the question, have they or have they not responded to the standard agents? And what has their response been? Should they be on glucose monitoring? How bad is their diabetes based on some laboratory parameters, et cetera, et cetera. So that's a very different information rich, electronic medical record rich mechanism to understand how to develop medicines. One lies, the first lies way in the discovery end of the spectrum. The second lies way in the clinical trials and human drug exposure end of the spectrum. And of course, there are things in the middle that I haven't iterated, but those are the two really broad categories where one can imagine AI making a difference and to be fair through various efforts, I'm working on both of those, the two end spectrum.
A.I. and Cancer
Eric Topol (08:34):
Well, let's drill down a bit more on the person individual informatics for a moment, since you're an oncologist, and the way we screen for cancer today is completely ridiculous by age only. But if you had a person's genome sequence, polygenic risk scores for cancers and all the other known data that, for example, the integrity of their immune system response, environmental exposures, which we'll talk about in a moment more, wouldn't we do far better for being able to identify high risk people and even preventing cancer in the future?
Sid Mukherjee (09:21):
So I have no doubt whatsoever that more information that we can analyze using intelligent platforms. And I'm saying all of these words are relevant, more information analyzed through intelligent platforms. More information by itself is often useless. Intelligent platforms without information by themselves are often useless, but more information with intelligent platforms, that combination can be very useful. And so, one use case of that is just to give you one example, there are several patients, women who have a family history of breast cancer, but who have no mutations in the known single monogenic breast cancer risk genes, BRCA1, BRCA2, and a couple of others. Those patients can be at a high a risk of breast cancer as patients who have BRCA1 and BRCA2. It's just that their risk is spread out through not one gene but thousands of genes. And those patients, of course have to be monitored and their risk is high, and they need to understand what the risk is and how to manage it.
(10:57):
And that's where AI can, and first of all, informatics and then AI can play a big difference because we can understand how to manage those patients. They used to be called, this is kind of, I don't mean this lightly, but they used to be called BRCA3 because they didn't have BRCA1, they didn't have BRCA2, but they had a constellation of genes, not one, not two, but thousands of genes that would increase their risk of breast cancer just a little bit. I often describe these as nudge genes as opposed to shove genes. BRCA1 and BRCA2 are shoved genes. They shove you into having a high risk of breast cancer. But you can imagine that there are nudge genes as well in which they, in which a constellation of not one, not two, not three, but a thousand genetic variations, give a little push each one, a little push towards having a higher risk of breast cancer.
(12:09):
Now, the only way to find these nudge genes is by doing very clever informatic studies, some of which have been done in breast cancer, ovarian cancer, cardiovascular diseases, other diseases where you see these nudge effects, small effects of a single gene, but accumulated across a thousand, 2000, 3000 genes, an effect that's large enough that it's meaningful. And I think that we need to understand those. And once we understand them, I think we need to understand what to do with these patients. Do we screen them more assertively? Do we recommend therapies? You can get more aggressive, less aggressive, but of course that demands clinical trials and a deeper understanding of the biology of what happens.
A.I. And Longevity
Eric Topol (13:10):
Right, so your point about the cumulative effects of small variants, hundreds and hundreds of these variants being equivalent potentially, as we've seen across many diseases, it's really important and you're absolutely right about that. And I've been pushing for trying to get these polygenic risk scores into clinical routine use, and hopefully we're getting closer to that. And that's just as you say, just one layer of thi
Holden Thorp: Straight Talk from the Editor-in-Chief of the Science family of journals
1h 0m · PublishedThere was so much to talk about—this is the longest Ground Truths podcast yet. Hope you’ll find it as thought-provoking as I did!
Transcript, with audio and external links, edited by Jessica Nguyen, Producer for Ground Truths
Video and audio tech support by Sinjun Balabanoff, Scripps Research
Eric Topol (00:00:05):
This is Eric Topol from Ground Truths, and I am delighted to have with me Holden Thorp, who is the Editor-in-Chief of the Science journals. We're going to talk about Science, not just the magazine journal, but also science in general. This is especially appropriate today because Holden was just recognized by STAT as one of the leaders for 2024 because of his extraordinary efforts to promote science integrity, so welcome Holden.
Holden Thorp (00:00:36):
Thanks Eric, and if I remember correctly, you were recognized by STAT in 2022, so it's an honor to join a group that you're in anytime, that's for sure, and great to be on here with you.
Eric Topol (00:00:47):
Well, that's really kind to you. Let's start off, I think with the journal, because I know that consumes a lot of your efforts and you have five journals within science.
Holden Thorp (00:01:02):
Oh, we have six.
Eric Topol (00:01:03):
Oh six, I'm sorry, six. There's Science, the original, and then five others. Can you tell us what it's like to oversee all these journals?
Overseeing the Science Journals
Holden Thorp (00:01:16):
Yeah, we're a relatively small family compared to our commercial competitors. I know you had Magdalena [Skipper]on and Nature has I think almost ninety journals, so six is pretty small. In addition to Science, which most people are familiar with, we have Science Advances, which also covers all areas of science and is larger and is a gold open access journal and also is overseen by academic editors, not professional editors. All of our other journals are overseen by professional editors. And then the other four are relatively small and specialized areas, and probably people who listen to you and follow you would know about Science Translational Medicine, Science Immunology, Science Signaling and then we also have a journal, Science Robotics which is something I knew nothing about and I learned a lot. I've learned a lot about robotics and the culture of people who work there interacting with them.
Holden Thorp (00:02:22):
So we have a relatively small family. There's only 160 people who work for me, which is manageable. I mean that sounds like a lot, but in my previous jobs I was a provost and a chancellor, and I had tens of thousands of people, so it's really fun for me to have a group where I at least have met everybody who works for me. We're an outstanding set of journals, so we attract an outstanding group of professionals who do all the things that are involved in all this, and it's really, really fun to work with them. At Science, we don't just do research papers, although that's a big, and probably for your listeners the biggest part of what we do. But we also have a news and commentary section and the news section is 30 full-time and many freelancers around the world really running the biggest general news operation for science that there is. And then in the commentary section, which you're a regular contributor for us in expert voices, we attempt to be the best place in the world for scientists to talk to each other. All three of those missions are just really, really fun for me. It's the best job I've ever had, and it's one I hope to do for many years into the future.
Eric Topol (00:03:55):
Well, it's extraordinary because in the four and a half years I think it's been since you took the helm, you've changed the face of Science in many ways. Of course, I think the other distinction from the Nature Journals is that it's a nonprofit entity, which shows it isn't like you're trying to proliferate to all sorts of added journals, but in addition, what you've done, at least the science advisor and the science news and all these things that come out on a daily basis is quite extraordinary as we saw throughout the pandemic. I mean, just reporting that was unparalleled from, as you say, all points around the world about really critically relevant topics. Obviously it extends well beyond the concerns of the pandemic. It has a lot of different functions, but what I think you have done two major things, Holden. One is you medicalized it to some extent.
Eric Topol (00:04:55):
A lot of people saw the journal, particularly Science per se, as a truly basic science journal. Not so much applied in a medical sphere, but these days there's more and more that would be particularly relevant to the practice of medicine, so that's one thing. And the other thing I wanted you to comment on is you're not afraid to speak out and as opposed to many other prior editors who I followed throughout my career at Science, there were pretty much the politically correct type and they weren't going to really express themselves, which you are particularly not afraid of. Maybe you could comment about if you do perceive this medicalization of science to some extent, and also your sense of being able to express yourself freely.
Capturing the Breakthroughs in Structural Biology
Holden Thorp (00:05:48):
Yeah, well, you're kind to say both of those things are certainly things we have worked at. I mean, I do come from a background, even though I'm trained as a chemist, most of what I did towards the latter end of my career, I mean, I did very basic biochemistry when I was a researcher, but the last part of my research career I worked in on development of a drug called Vivjoa, which is an alternative to the fluconazole family that doesn't have the same toxicity and is currently on the market for chronic yeast infection and hopefully some other things in the future when we can get some more clinical trials done.
Holden Thorp (00:06:35):
And I've hung around biotech startups and drug development, so it is part of the business that I knew. I think the pandemic really gave us an opening because Valda Vinson, who's now the Executive Editor and runs all of life sciences for us and policies for the journal, she was so well known in structural biology that most of the first important structures in Covid, including the spike protein, all came to us. I mean, I remember crystal clear February of 2020, she came in my office and she said, I got the structure of the spike protein. And I said, great, what's the spike protein? Turned out later became the most famous protein in the world, at least temporarily. Insulin may be back to being the most famous protein now, but spike protein was up there. And then that kind of cascaded into all the main protease and many of the structures that we got.
Holden Thorp (00:07:45):
And we seized on that for sure, to kind of broaden our focus. We had the Regeneron antibodies, we had the Paxlovid paper, and all of that kind of opened doors for us. And we've also, now we have two clinical editors at Science, Priscilla Kelly and Yevgeniya Nusinovich, and then the Insights section, somebody that you work with closely, Gemma Alderton, she is very fluent in clinical matters. And then of course we've had Science Translational Medicine and we seek continue to strengthen that. Science Immunology was very much boosted by Covid and actually Science Immunology is now, I think probably if you care about impact factors, the second highest specialized immunology journal after Immunity. I've put some emphasis on it for sure, but I think the pandemic also really helped us. As far as me speaking out, a lot of people maybe don't remember, but Don Kennedy, who was the editor in the early 2000s who had been the Stanford president, he was similarly outspoken.
Confronting Controversies
Holden Thorp (00:09:15):
It's funny, sometimes people who disagree with me say, well, Don Kennedy would never say anything like that. And then I can dig up something that Don Kennedy said that's just as aggressive as what I might've said. But you're right, Bruce Alberts was very focused on education, and each one of us has had our own different way of doing things. When Alan Leshner hired me and Sudip Parikh reinforced this when he came on, I mean, he wanted me to liven up the editorial page. He explicitly told me to do that. I may have done more of it than he was expecting, but Alan and Sudip both still remain very supportive of that. I couldn't do what I do without them and also couldn't do it without Lisa Chong, who makes all my words sound so much better than they are when I start. And yeah, it kind of fed on itself.
Holden Thorp (00:10:21):
It started with the pandemic. I think there was an inflection when Trump first said that Covid was just the flu, and when he said some really ridiculous things about the vaccine, and that's where it started. I guess my philosophy was I was thinking about people who, they've got a spouse at home whose job might be disrupted. They got children they've got who are out of school, and somehow they managed to get themselves to the lab to work on our vaccine or some other aspect of the pandemic to try to help the world. What would those people want their journal to say when they came home and turned the news on and saw all these politicians saying all this ridiculous stuff? That was really the sort of mantra that I had in my head, and that kind of drove it. And now I think we've sort of established the fact that it's okay to comment on things that are going on in the world. We're editorially independent, Sudip and the AAAS board, treat us as being editorially independent. I don't take tha
Daphne Koller: The Convergence of A.I. and Digital Biology
35m · PublishedTranscript
Eric Topol (00:06):
Well, hello, this is Eric Topol with Ground Truths and I am absolutely thrilled to welcome Daphne Koller, the founder and CEO of insitro, and a person who I've been wanting to meet for some time. Finally, we converged so welcome, Daphne.
Daphne Koller (00:21):
Thank you Eric. And it's a pleasure to finally meet you as well.
Eric Topol (00:24):
Yeah, I mean you have been rocking everybody over the years with elected to the National Academy of Engineering and Science and right at the interface of life science and computer science and in my view, there's hardly anyone I can imagine who's doing so much at that interface. I wanted to first start with your meeting in Davos last month because I kind of figured we start broad AI rather than starting to get into what you're doing these days. And you had a really interesting panel [←transcript] with Yann LeCun, Andrew Ng and Kai-Fu Lee and others, and I wanted to get your impression about that and also kind of the general sense. I mean AI is just moving it at speed, that is just crazy stuff. What were your thoughts about that panel just last month, where are we?
Video link for the WEF Panel
Daphne Koller (01:25):
I think we've been living on an exponential curve for multiple decades and the thing about exponential curves is they are very misleading things. In the early stages people basically take the line between whatever we were last year, and this year and they interpolate linearly, and they say, God, things are moving so slowly. Then as the exponential curve starts to pick up, it becomes more and more evident that things are moving faster, but it’s still people interpolate linearly and it's only when things really hit that inflection point that people realize that even with the linear interpolation where we'll be next year is just mind blowing. And if you realize that you're on that exponential curve where we will be next year is just totally unanticipatable. I think what we started to discuss in that panel was, are we in fact on an exponential curve? What are the rate limiting factors that may or may not enable that curve to continue specifically availability of data and what it would take to make that curve available in areas outside of the speech, whatever natural language, large language models that exist today and go far beyond that, which is what you would need to have these be applicable to areas such as biology and medicine.
Daphne Koller (02:47):
And so that was kind of the message to my mind from the panel.
Eric Topol (02:53):
And there was some differences in opinion, of course Yann can be a little strong and I think it was good to see that you're challenging on some things and how there is this “world view” of AI and how, I guess where we go from here. As you mentioned in the area of life science, there already had been before large language models hit stride, so much progress particularly in imaging cells, subcellular, I mean rare cells, I mean just stuff that was just without any labeling, without fluorescein, just amazing stuff. And then now it's gone into another level. So as we get into that, just before I do that, I want to ask you about this convergence story. Jensen Huang, I'm sure you heard his quote about biology as the opportunity to be engineering, not science. I'm sure if I understand, not science, but what about this convergence? Because it is quite extraordinary to see two fields coming together moving at such high velocity.
"Biology has the opportunity to be engineering not science. When something becomes engineering not science it becomes...exponentially improving, it can compound on the benefits of previous years." -Jensen Huang, NVIDIA.
Daphne Koller (04:08):
So, a quote that I will replace Jensen's or will propose a replacement for Jensen's quote, which is one that many people have articulated, is that math is to physics as machine learning is to biology. It is a mathematical foundation that allows you to take something that up until that point had been kind of mysterious and fuzzy and almost magical and create a formal foundation for it. Now physics, especially Newtonian physics, is simple enough that math is the right foundation to capture what goes on in a lot of physics. Biology as an evolved natural system is so complex that you can't articulate a mathematical model for that de novo. You need to actually let the data speak and then let machine learning find the patterns in those data and really help us create a predictability, if you will, for biological systems that you can start to ask what if questions, what would happen if we perturb the system in this way?
The Convergence
Daphne Koller (05:17):
How would it react? We're nowhere close to being able to answer those questions reliably today, but as you feed a machine learning system more and more data, hopefully it'll become capable of making those predictions. And in order to do that, and this is where it comes to this convergence of these two disciplines, the fodder, the foundation for all of machine learning is having enough data to feed the beast. The miracle of the convergence that we're seeing is that over the last 10, 15 years, maybe 20 years in biology, we've been on a similar, albeit somewhat slower exponential curve of data generation in biology where we are turning it into a quantitative discipline from something that is entirely observational qualitative, which is where it started, to something that becomes much more quantitative and broad based in how we measure biology. And so those measurements, the tools that life scientists and bioengineers have developed that allow us to measure biological systems is what produces that fodder, that energy that you can then feed into the machine learning models so that they can start making predictions.
Eric Topol (06:32):
Yeah, well I think the number of layers of data no less what's in these layers is quite extraordinary. So some years ago when all the single cell sequencing was started, I said, well, that's kind of academic interest and now the field of spatial omics has exploded. And I wonder how you see the feeding the beast here. It's at every level. It's not just the cell level subcellular and single cell nuclei sequencing single cell epigenomics, and then you go all the way to these other layers of data. I know you plug into the human patient side as well as it could be images, it could be past slides, it could be the outcomes and treatments and on and on and on. I mean, so when you think about multimodal AI, has anybody really done that yet?
Daphne Koller (07:30):
I think that there are certainly beginnings of multimodal AI and we have started to see some of the benefits of the convergence of say, imaging and omics. And I will give an example from some of the work that we've recently distributed on a preprint server work that we did at insitro, which took imaging data from standard histopathology slides, H&E slides and aligned them with simple bulk RNA-Seq taken from those same tumor samples. And what we find is that by training models that translate from one to the other, specifically from the imaging to the omics, you're able to, for a fairly large fraction of genes, make very accurate predictions of gene expression levels by looking at the histopath images alone. And in fact, because many of the predictions are made at the tile level, not at the entire slide level, even though the omics was captured in bulk, you're able to spatially resolve the signal and get kind of like a pseudo spatial biology just by making predictions from the H&E image into these omic modalities.
Multimodal A.I. and Life Science
Daphne Koller (08:44):
So there are I think beginnings of multimodality, but in order to get to multimodality, you really need to train on at least some data where the two modalities are simultaneously. And so at this point, I think the rate limiting factor is more a matter of data acquisition for training the models. It is for building the models themselves. And so that's where I think things like spatial biology, which I think like you are very excited about, are one of the places where we can really start to capture these paired modalities and get to some of those multimodal capabilities.
Eric Topol (09:23):
Yeah, I wanted to ask you because I mean spatial temporal is so perfect. It is two modes, and you have as the preprint you refer to and you see things like electronic health records in genomics, electronic health records in medical images. The most we've done is getting two modes of data together. And the question is as this data starts to really accrue, do we need new models to work with it or do you actually foresee that that is not a limiting step?
Daphne Koller (09:57):
So I think currently data availability is the most significant rate limiting step. The nice thing about modern day machine learning is that it really is structured as a set of building blocks that you can start to put together in different ways for different situations. And so, do we have the exact right models available to us today for these multimodal systems? Probably not, but do we have the right building blocks that if we creatively put them together from what has already been deployed in other settings? Probably, yes. So of course there's still a model exploration to be done and a lot of creativity in how these building blocks should be put together, but I think we have the tools available to solve these problems. What we really need is first I think a really significant data acquisition effort. And the other thing that we need, which is also something that has been a priority for us at insitro, is the right mix of people to be put together so that you can, because what
Coleen Murphy: The Science of Aging and Longevity
44m · Published“A few years ago, I might have chuckled at the naiveté of this question, but now it's not so crazy to think that we will be able to take some sort of medicine to extend our healthy lifespans in the foreseeable future.”—Coleen Murphy
Transcript with external links
Eric Topol (00:06):
Hello, this is Eric Topol from Ground Truths, and I'm just so delighted to have with me Professor Coleen Murphy, who has written this exceptional book, How We Age: The Science of Longevity. It is a phenomenal book and I'm very eager to discuss it with you, Coleen.
Coleen Murphy (00:25):
Thanks for having me on.
Eric Topol (00:27):
Oh yeah. Well, just so everyone who doesn't know Professor Murphy, she's at Princeton. She's the Richard Fisher Preceptor in Integrative Genomics, the Lewis-Sigler Institute for Integrative Genomics at Princeton, and director of the Paul Glenn Laboratories for Aging Research. Well, obviously you've been in this field for decades now, even though you're still very young. The classic paper that I can go back to would be in Nature 2003 with the DAF-16 and doubling the lifespan of C. elegans or better known as a roundworm. Would that be the first major entry you had?
Coleen Murphy (01:17):
Yeah, that was my postdoctoral work with Cynthia Kenyon.
Eric Topol (01:20):
Right, and you haven't stopped since you've been on a tear and you’ve put together a book which has a hundred pages of references in a small font. I don't know what the total number is, but it must be a thousand or something.
Coleen Murphy (01:35):
Actually, it's just under a thousand. That's right.
Eric Topol (01:37):
That's a good guess.
Coleen Murphy (01:38):
Good guess. Yeah.
Eric Topol (01:39):
So, because I too have a great interest in this area, I found just the resource that you've put together as extraordinary in terms of the science and all the work you've put together. What I was hoping to do today is to kind of take us through some of the real exciting pathways because there's a sentence in your book, which I thought was really kind of nailed it, and it actually is aligned with my sense. Obviously don't have the expertise by any means that you do here but it says, “A few years ago, I might have chuckled at the naivety of this question, but now it's not so crazy to think that we will be able to take some sort of medicine to extend our healthy lifespans in the foreseeable future.” That's a pretty strong statement for a person who's deep into the science. First I thought we'd explore healthy aging health span versus lifespan. Can you differentiate that as to your expectations?
Coleen Murphy (02:54):
So, I think most people would agree that they don't want to live necessary super long. What they really want to do is live a healthy life as long as they can. I think that a lot of people also have this fear that when we talk about extending lifespan, that we're ignoring that part. And I do want to assure everyone that the people in the researchers in the aging field are very much aware of this issue and have, especially in the past decade, I think put a real emphasis on this idea of quality of life and health span. What's reassuring is actually that many of the mechanisms that extend lifespan in all these model organisms also extend health span as well and so I don't think we're going to, they're not diametrically opposed, like we'll get to a healthier quality of life, I think in these efforts to extend lifespan as well.
Eric Topol (03:50):
Yeah, I think that's important that you're bringing that up, which is there's this overlap, like a Venn diagram where things that do help with longevity should help with health span, and we don't necessarily have to follow as you call them the immoralists, as far as living to 190 or whatever year. Now, one of the pathways that's been of course a big one for years and studied in multiple species has been caloric restriction. I wonder if you could talk to that and obviously there's now mimetics that could simulate that so you wouldn't have to go through some major dietary starvation, if you will. What are your thoughts on that pathway?
Coleen Murphy (04:41):
Yeah, actually I'm really glad you brought up mimetics because often the conversation starts and ends with you should eat less. I think that is a really hard thing for a lot of people to do. So just for the background, so dietary restriction or caloric restriction, the idea is that you would have to take in up to 30% less than your normal intake in order to start seeing results. When we've done this with laboratory animals of all kinds, this works from yeast all the way up through mice, actually primates, in fact, it does extend lifespan and in most metrics of health span the quality of life, it does improve that as well. On the other hand, I think psychologically it's really tough to not eat enough and I think that's a part that we kind of blindly ignore when we talk about this pathway.
Coleen Murphy (05:30):
And of course, if we gave any of those animals the choice of whether they want to start eating more, they would. So, it's like that's not the experiment we ever hear about. And so, the idea for studying this pathway isn't just to say, okay, this works and now we know how it works, but as you pointed out, mimetics, so can we target the molecules in the pathway so that we can help people achieve the benefits of caloric restriction without necessarily having to do the kind of awful part of restriction? I think that's really cool, and especially it might be very good for people who are undergoing certain, have certain diseases or have certain impairments that it might make it difficult ever to do dietary restrictions, so I think that's a really great thing that the field is kind of getting towards now.
Eric Topol (06:15):
And I think in fact, just today, it's every day there's something published now. Just today there was a University of Southern California study, a randomized study report comparing plant-based fasting-mimicking diet versus controlled diet, and showed that many metabolic features were improved quite substantially and projected that if you stayed on that diet, you'd gain two and a half years of healthy aging or that you would have, that's a bit of an extrapolation, but quite a bit of benefit. Now, what candidates would simulate caloric restriction? I mean, what kind of molecules would help us do that? And by the way, in the book you mentioned that the price to pay is that the brain slows down with caloric restrictions.
Coleen Murphy (07:10):
There's at least one study that shows that.
Coleen Murphy (07:13):
Yeah, so it's good to keep in mind. One of the big things that is being looked at as rapamycin, looking at that TOR pathway. So that's being explored as one of these really good mimetics. And of course, you have things that are analogs of that, so rapalogs, and so people are trying to develop drugs that mimic that, do the same kind of thing without probably some of the side effects that you might see with rapamycin. Metformin is another one, although it's interesting when you talk to people about metformin who work on it, it's argued about what is exactly the target of metformin. There's thought maybe also acts in the TOR pathway could affect complex one of mitochondria. Some of the things we know that they work, and we don't necessarily know how they work. And then of course there's new drugs all the time where people are trying to develop to other target, other molecules. So, we'll see, but I think that the idea of mimetics is actually really good, and that part of the field is moving forward pretty quickly. This diet that you did just mention, it is really encouraging that they don't have to take a drug if you don't want to. If you eat the right kind of diet, it could be very beneficial.
Eric Topol (08:20):
Yeah, no, it was interesting. I was looking at the methods in that USC paper and they sent them a box of stuff that they would eat for three cycles, multiple weeks per cycle. It was a very interesting report, we'll link to that. Before we leave the caloric restriction and these mTOR pathway, you noted in the book that there some ongoing trials like PEARL, I looked that up and they finished the trial, but they haven't reported it and it's not that large. And then there's the FAME trial with metformin. I guess we'll get a readout on these trials in the not-too-distant future. Right?
Coleen Murphy (08:57):
Yeah, that's the hope that especially with the Metformin trial, which I think is going to be really large the FAME trial, that just to give the listeners a little background, one of the efforts in the field is not just to show that something works, but also to convince the FDA that aging could be a pharmaceutical, a disease that we might want to have interventions for. And to do that, we need to figure out the right way to do it. We can't do 30-year studies of safety and things to make sure that something's good, but maybe there are reasonable biomarkers that would tell us whether people are going to live a long time. And so, if we can use some of those things or targeting age-related diseases where we can get a faster readout as well. Those are reasonable things that companies could do that would help us to really confirm or maybe rule out some of these pharmaceuticals as effective interventions. I think that would be really great for consumers to know, is this thing really going to do good or not? And we just don't have that right now in the field. We have a lot of people saying something will work and it might and the studies in the lab, but when we get to humans, we really need more clinical studies to really tell us that things are going to be effect
Michelle Monje: The Brain in Long Covid and Cancer
43m · PublishedTranscript with audio and relevant external links, recorded on 6 Feb 2024
Eric Topol (00:05):
Hello, this is Eric Topol with Ground Truths, and I have a remarkable guest with me today, Professor Michelle Monje, who is from Stanford, a physician-scientist there and is really a leader in neuro-oncology, the big field of cancer neuroscience, neuroinflammation, and she has just been rocking it recently with major papers on these fields, no less her work that's been on a particular cancer, brain cancer in kids that we'll talk about. I just want to give you a bit of background about Michelle. She is a National Academy of Medicine member, no less actually a National Academy of Medicine awardee with the French Academy for the Richard Lounsbery Award, which is incredibly prestigious. She received a Genius grant from the MacArthur Foundation and is a Howard Hughes Medical Institute (HHMI) scholar, so she is just an amazing person who I'm meeting for the first time. Michelle, welcome.
Michelle Monje (01:16):
Thank you. So nice to join you.
Long Covid and the Brain
Eric Topol (01:18):
Well, I just am blown away by the work that you and your colleagues have been doing and it transcends many different areas that are of utmost importance. Maybe we can start with Long Covid because that's obviously such a big area. Not only have you done work on that, but you published an amazing review with Akiko Iwasaki, a friend of mine, that really went through all the features of Long Covid. Can you summarize your thoughts about that?
Michelle Monje (01:49):
Yeah, and specifically we focused on the neurobiology of Long Covid focusing on the really common syndrome of cognitive impairment so-called brain fog after Covid even after relatively mild Covid. There has been this, I think really important and exciting, really explosion of work in the last few years internationally trying to understand this in ways that I am hopeful will be beneficial to many other diseases of cognition that occur in the context of other kinds of infections and other kinds of immune challenges. But what is emerging from our work and from others is that inflammation, even if it doesn't directly initially involve the nervous system, can very profoundly affect the nervous system and the mechanisms by which that can happen are diverse. One common mechanism appears to be immune challenge induced reactivity of an innate immune cell in the nervous system called microglia. These microglia, they populate the nervous system very early in embryonic development.
(02:58):
And their job is to protect the nervous system from infection, but also to respond to other kinds of toxic and infectious and immune challenges. They also play in healthy conditions, really important roles in neurodevelopment and in neuroplasticity and so they're multifaceted cells and this is some population of those cells, particularly in the white matter in the axon tracks that are exquisitely sensitive it seems to various kinds of immune challenges. So even if there's not a direct nervous system insult, they can react and when they react, they stop doing their normal helpful jobs and can dysregulate really important interactions between other kinds of cells in the brain like neurons and support cells for those neurons like oligodendrocytes and astrocytes. One common emerging principle is that microglial reactivity triggered by even relatively mild Covid occurring in the respiratory system, not directly infecting the brain or other kinds of immune challenges can trigger this reactivity of microglia and consequently dysregulate the normal interactions between cells and the brain.
(04:13):
So important for well-tuned and optimal nervous system function. The end product of that is dysfunction and cognition and kind of a brain fog impairment, attention, memory, ability to multitask, impaired speed of information processing, but there are other ways that Covid can influence the nervous system. Of course there can be direct infection. We don't think that that happens in every case. It may not happen even commonly, but it certainly can happen. There is a clear dysregulation of the vasculature, the immune response, and the reaction to the spike protein of Covid in particular can have very important effects on the vessels in the nervous system and that can trigger a cascade of effects that can cause nervous system dysregulation and may feed directly into that reactivity of the microglia. There also can be reactivation of other infections previous, for example, herpes virus infections. EBV for example, can be reactivated and trigger a new immune challenge in the context of the immune dysregulation that Covid can induce.
(05:21):
There also can be autoimmunity. There are many, we're learning all the different ways Covid can affect the nervous system, but autoimmunity, there can be mimicry of some of the antigens that Covid presents and unfortunate autoimmunity against nervous system targets. Then finally in severe Covid where there is cardiopulmonary compromise, where there is hypoxia and multi-organ damage, there can be multifaceted effects on the nervous system in severe disease. So many different ways, and probably that is not a comprehensive list. It is certainly not a mutually exclusive list. Many of these interactions can happen at the same time in the same individual and in different combinations but we're beginning to wrap our arms around all the different ways that Covid can influence the nervous system and cause this fairly consistent syndrome of impaired attention, memory, multitasking, and executive functions.
Homology with Chemo Brain
Eric Topol (06:23):
Yeah, well there's a lot there that you just summarized and particularly you highlighted the type of glia, the microglia that appear to be potentially central at least a part of the story. You also made analogy to what you've seen with chemotherapy, chemo brain. Maybe you could elaborate on that.
Michelle Monje (06:42):
Yeah, absolutely. So I've been studying the cognitive impairment that can happen after cancer therapies including chemotherapy, but also radiation and immunotherapy. Each time we develop a new model and dig in to understand what's going on and how these cancer therapies influence the nervous system, microglia emerge as sort of the unifying principle, microglial reactivity, and the consequences of that reactivity on other cell types within the nervous system. And so, understanding that microglia and their reactive state to toxic or immune challenges was central to chemotherapy induced cognitive impairment, at least in preclinical models in the laboratory and confirm by human tissue studies. I worried at the very beginning of the pandemic that we might begin to see something that looks a lot like chemotherapy induced cognitive impairment, this syndrome that is characterized by impaired attention, memory, executive function, speed of information processing and multitasking. When just a few months into the pandemic, people began to flood neurologists’ office complaining of exactly this syndrome. I felt that we needed to study it and so that was the beginning of what has become a really wonderful collaboration with Akiko Iwasaki. I reached out to her, kind of cold called her in the midst of the deep Covid shutdown and in 2020 and said, hey, I have this idea, would you like to work with me? She's as you know, just a thought leader in Covid biology and she's been an incredibly wonderful and valuable collaborator along the way in this.
Eric Topol (08:19):
Well, the two of you pairing up is kind of, wow, that's a powerful combination, no question. Now, I guess the other thing I wanted to get at is there've been many other studies that have been looking at Long Covid, how it affects the brain. The one that's frequently cited of course is the UK Biobank where they had CT or MRI scans before in people fortunately, and then once they had Covid or didn't get Covid and it had a lot of worrisome findings including atrophy and then there are others that in terms of this niche of where immune cells can be in the meninges, in the bone marrow or the skull of the brain. Could you comment on both those issues because they've been kind of coming back to haunt us in terms of the more serious potential effects of Covid on the brain?
Michelle Monje (09:20):
Yeah, absolutely and I will say that I think all of the studies are actually quite parsimonious. They all really kind of point towards the same biology, examining it at different levels. And so that UK Biobank study was so powerful because in what other context would someone have MRI scans across the population and cognitive testing prior to the Covid pandemic and then have paired same individual tests after a range of severity of Covid infection so it was just an incredibly important data set with control individuals in the same cohort of people. This longitudinal study has continued to inform us in such important ways and that study found that there were multiple findings. One is that there appears to be a small but significant atrophy in the neocortex. Two that there are also abnormalities in major white matter tracts, and three, that there is particular pathology within the olfactory system.
(10:30):
And we know that Covid induces as a very common early symptom, this loss of smell. Then together with those structural findings on MRI scans that individuals even with relatively mild acute disease, exhibited long-term deficits in cognitive function. That fits with some beautiful epidemiological studies that have been done across many thousands of individuals in multiple different geographic populations. Underscoring this consistent finding that Covid can induce lasting cognitive changes and as we begin to understand that biology
Jim Collins: Discovery of the First New Structural Class of Antibiotics in Decades, Using A.I.
28m · PublishedJim Collins is one of the leading biomedical engineers in the world. He’s been elected to all 3 National Academies (Engineering, Science, and Medicine) and is one of the founders of the field of synthetic biology. In this conversation, we reviewed the seminal discoveries that he and his colleagues are making at the Antibiotics-AI Project at MIT.
Recorded 5 February 2024, transcript below with audio links and external links to recent publications
Eric Topol (00:05):
Hello, it's Eric Topol with Ground Truths, and I have got an extraordinary guest with me today, Jim Collins, who's the Termeer Professor of Medical Engineering at MIT. He also holds appointments at the Wyss Institute and the Broad Institute. He is a biomedical engineer who's been making exceptional contributions and has been on a tear lately, especially in the work of discovery of very promising, exciting developments in antibiotics. So welcome, Jim.
Jim Collins (00:42):
Eric, thanks for having me on the podcast.
Eric Topol (00:44):
Well, this was a shock when I saw your paper in Nature in December about a new structure class of antibiotics, the one from 1962 to 2000. It took 38 years, and then there was another one that took 24 years yours, the structural antibiotics. Before I get to that though, I want to go back just a few years to the work you did published in Cell with halicin, and can you tell us about this? Because when I started to realize what you've been doing, what you've been chipping away here, this was a drug you found, halicin, as I can try to understand, it works against tuberculosis, c. difficile, enterobacter that are resistant, acinetobacter that are resistant. I mean, this is, and this is of course in mice models. Can you tell us how did you make that discovery before we get into I guess what's called the Audacious Project?
Jim Collins (01:48):
Yeah, sure. It's actually a fun story, so it is origins go broadly to institute wide event at MIT, so MIT in 2018 launched a major campus-wide effort focused on artificial intelligence. The institute, which had played a major role in the first wave of AI in the 1950s, 1960s, and a major wave in the second wave in the 1980s found itself kind of at the wheel in this third wave involving big data and deep learning and looked to correct that and to correct it the institute had a symposium and I had the opportunity to sit next to Regina Barzilay, one of our faculty here at MIT who specializes in AI and particularly AI applied to biomedicine and we really hit it off and realized we had interest in applying AI to drug discovery. My lab had focused on antibiotics to then close to 15 years, but primarily we're using machine learning and network biology to understand the mechanism of action of antibiotics and how resistance arise with the goal of boosting what we already had, with Regina we saw there was an opportunity to see if we could use deep learning to get after discovery.
(02:55):
And notably, as you kind of alluded in your introduction, there's really been a discovery void and the golden age of discovery antibiotics was in the forties, fifties and sixties before I was born and before you had the genomic revolution, the biotech revolution, AI revolution. Anyways, we got together with our two groups, and it was an unfunded project and we kind of cobbled together very small training set of 2,500 compounds that included 1,700 FDA approved drugs and 800 natural compounds. In 2018, 2019, when you started this, if you asked any AI expert should you initiate that study, they would say absolutely not, there's going to be two big data. The idea of these models are very data hungry. You need a million pictures of a dog, a million pictures of a cat to train a model to differentiate between the cat and the dog, but we ignored the naysayers and said, okay, let's see what we can do.
(03:41):
And we apply these to E. coli, so a model pathogen that's used in labs but is also underlies urinary tract infections. So it’s a look to see which of the molecules inhibited growth of the bacteria as evidence for antibacterial activity and we could have measured and we quantified each of their effects, but because we had so few compounds, we just discretized instead, if you inhibited at least 80% of the growth you were antibacterial, and if you didn't achieve that, you weren't antibacterial zero in ones. We then took the structure of each molecule and trained a deep learning model, specifically a graphical neural net that could look at those structures, bond by bond, substructure by substructure associated with whatever features you look to train with. In our case, making for good antibiotic, not for good antibiotic. We then took the train model and applied it to a drug repurposing hub as part of the Broad Institute that consists of 6,100 molecules in various stages of development as a new drug.
(04:40):
And we asked the model to identify molecules that can make for a good antibiotic but didn't look like existing antibiotics. So part of the discovery void has been linked to this rediscovery issue we have where we just keep discovering quinolones like Cipro or beta-lactams like penicillin. Well, anyways, from those criteria as well as a small tox model, only one molecule came out of that, and that was this molecule we called halicin, which was named after HAL, the killing AI computer system from 2001 Space Odyssey. In this case, we don't want it to kill humans, we want it to kill bacteria and as you alluded, it turned out to be a remarkably potent novel antibiotic that killed off multi-drug resistant extensively drugs, a pan-resistant bacteria went after to infections. It was affected against TB, it was affected against C. diff and acinetobacter baumannii and acted to a completely new mechanism of action.
(05:33):
And so we were very excited to see how AI could open up possibilities and enable one to explore chemical spaces in new and different ways. We took them model, then applied it to a very large chemical library of 1.5 billion molecules, looked at a subset of about 110 million that would be impossible for any grad student, any lab really to look at that experimentally but we looked at it in a model computer system and in three days could screen those 110 million molecules and identified several new additional candidates, one which we call salicin, which is the cousin of halicin that similes broad spectrum and acts to a novel mechanism of action.
Eric Topol (06:07):
So before we go further with this initial burst of discovery, for those who are not used to deep neural networks, I think most now are used to the convolutional neural network for images, but what you use specifically here as you alluded to, were graph neural networks that you could actually study the binding properties. Can you just elaborate a little bit more about these GNN so that people know this is one of the tools that you used?
Jim Collins (06:40):
Yeah, so in this case, the underlying structure of the model can actually represent and capture a graphical structure of a molecule or it might be of a network so that the underlying structure itself of the model will also look at things like a carbon atom connects to an oxygen atom. The oxygen atom connects to a nitrogen atom and so when you think back to the chemical structures we learned in high school, maybe we learned in college, if we took chemistry class in college, it was actually a model that can capture the chemical structure representation and begin to look at sub aspects of it, associating different properties of it. In this case, again, ours was antibacterial, but it could be toxic, whether it's toxic against a human cell and the model, the train model, the graph neural model can now look at new structures that you input them and then make calculations on those bonds so a bond would be a connection between two atoms or substructures, be multiple bonds, interconnecting multiple atoms and assign it a score. Does it make, for example, in our case, for a good antibiotic.
Eric Topol (07:48):
Right. Now, what's also striking as you set up this collaboration that's interdisciplinary with Regina, who I know of her work through breast cancer AI and not through drug discovery and so this was, I think that new effort and this discovery led to this, I love the name of it, Audacious Project, right?
Jim Collins (08:13):
Right. Yeah, so a few points on the collaboration then I'll speak to Audacious Project. In addition to Regina, we also brought in Tommi Jaakkola, another AI faculty member and marvelous colleague here at MIT and really we've benefited from having outstanding young folks who were multilingual. We had very rich, deep trained grad students from ML on Regina and Tommi's side who appreciated the biology and we had very richly, deeply trained postdocs, Jon Stokes in particular from the microbiology side on my side, who could appreciate the machine learning and so they could speak across the divide. And so, as I look out in the next few decades in this exciting time of AI coming into biomedicine, I think the groups will make a difference of those that have these multilingual young trainees and two who are well set up to also inject human intelligence with machine intelligence.
(09:04):
Brings the Audacious Project. Now, prior to our publication of halicin, I was invited by the Audacious Project to submit a proposal, the Audacious Project is a new philanthropic effort run by TED, so the group that does the TED Talks that's run by Chris Anderson, so Chris had the idea that there was a need to bring together philanthropists around the world to go for a larger scale in a collective manner toward audacious projects. I pitched them on the idea that we could use AI to address the antibiotic resistance crisis. As
Ground Truths has 30 episodes in total of non- explicit content. Total playtime is 21:07:01. The language of the podcast is English. This podcast has been added on February 4th 2024. It might contain more episodes than the ones shown here. It was last updated on May 10th, 2024 01:42.