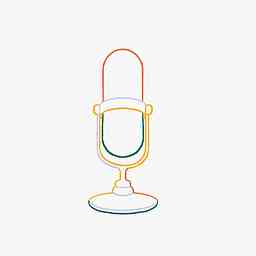
Ground Truths
by Eric Topol
Facts, data, and analytics about biomedical matters.
erictopol.substack.com
Copyright: Eric Topol
Episodes
Andrew Ng: On OpenAI's stormy times, AI regulation, education, and where we are headed for healthcare and beyond
32m · Published“A.I. is not the problem; it’s the solution.”—Andrew Ng at TED, 17 October 2023
Recorded 21 November 2023
Transcript with relevant links and links to audio file
Eric Topol (00:00):
Hello, it's Eric Topol with Ground Truths, and I'm really delighted to have with me Andrew Ng, who is a giant in AI who I've gotten to know over the years and have the highest regard. So Andrew, welcome.
Andrew Ng (00:14):
Hey, thanks Eric. It's always a pleasure to see you.
Eric Topol (00:16):
Yeah, we've had some intersections in multiple areas of AI. The one I wanted to start with is that you've had some direct healthcare nurturing and we've had the pleasure of working with Woebot Health, particularly with Alison Darcy, where the AI chatbot has been tested in randomized trials to help people with depression and anxiety. And, of course, that was a chatbot in the pre-transformer or pre-LLM era. I wonder if you could just comment about that as well as your outlook for current AI models in healthcare.
Andrew Ng (01:05):
So Alyson Darcy is brilliant. It's been such a privilege to work with her over the years. One of the exciting things about AI is a general purpose technology. It's not useful for one thing. And I think in healthcare and more broadly across the world, we're seeing many creative people use AI for many different applications. So I was in Singapore a couple months ago and I was chatting with some folks, Dean Chang and one of his doctors, Dr. M, about how they're using AI to read EHRs in a hospital in Singapore to try to estimate how long a patient's going to be in the hospital because of pneumonia or something. And it was actually triggering helpful for conversations where a doctor say, oh, I think this patient will be in for three days, but the AI says no, I'm guessing 15 days. And this triggers a conversation where the doctor takes a more careful look. And I thought that was incredible. So all around the world, many innovators everywhere, finding very creative ways to apply AI to lots of different problems. I think that's super exciting.
Eric Topol (02:06):
Oh, it's extraordinary to me. I think Geoff Hinton has thought that the most important application of current AI is in the healthcare/ medical sphere. But I think that the range here is quite extraordinary. And one of the other things that you've been into for all these years with Coursera starting that and all the courses for deep learning.AI —the democratization of knowledge and education in AI. Since this is something like all patients would want to look up on whatever GPT-X about their symptoms different than of course a current Google search. What's your sense about the ability to use generative AI in this way?
Andrew Ng (02:59):
I think that instead of seeing a doctor as a large language model, what's up with my symptoms, people are definitely doing it. And there have been anecdotes of this maybe saving a few people's lives even. And I think in the United States we're privileged to have some would say terrible, but certainly better than many other country’s healthcare system. And I feel like a lot of the early go-to market for AI enabled healthcare may end up being in countries or just places with less access to doctors. The definitely countries where you can either decide do you want to go see if someone falls sick? You can either send your kid to a doctor or you can have your family eat for the next two weeks, pick one. So with families made these impossible decisions, I wish we could give everyone in the world access to a great doctor and sometimes the alternatives that people face are pretty harsh. I think any hope, even the very imperfect hope of LLM, I know it sounds terrible, it will hallucinate, it will give bad medical advice sometimes, but is that better than no medical advice? I think there's really some tough ethical questions are being debated around the world right now.
Eric Topol (04:18):
Those hallucinations or confabulation, won't they get better over time?
Andrew Ng (04:24):
Yes, I think LLM technology is advanced rapidly. They still do hallucinate, they do still mix stuff up, but it turns out that I think people still have an impression of LLM technology from six months ago. But so much has changed in the last six months. So even in the last six months, it is actually much harder now to get an LMM, at least many of the public ones offered by launch companies. It's much harder now compared to six months ago to get it to give you deliberately harmful advice or if you ask it for detailed instructions on how to commit a crime. Six months ago it was actually pretty easy. So that was not good. But now it's actually pretty hard. It's not impossible. And I actually ask LLMs for strange things all the time just to test them. And yes, sometimes I can get them when I really try to do something inappropriate, but it's actually pretty difficult.
(05:13):
But hallucination is just a different thing where LLMs do mix stuff up and you definitely don't want that when it comes to medical advice. So it'll be an interesting balance I think of when should we use web search for trust authoritative sources. So if I have a sprained ankle, hey, let me just find a webpage on trust from a trusted medical authority on how to deal with sprained ankle. But there are also a lot of things where there is no one webpage that just gives me an answer. And then this is an alternative for generating a novel thing that's need to my situation. In non-healthcare cases, this has clearly been very valuable in just the healthcare, given the criticality of human health and human life. I think people are wrestling with some challenging questions, but hallucinations are slowly going down.
Eric Topol (05:59):
Well, hopefully they'll continue to improve on that. And as you pointed out the other guardrails that will help. Now that gets me to a little over a month ago, we were at the TED AI program and you gave the opening talk, which was very inspirational, and you basically challenged the critics of the negativism on AI with three basic issues: amplifying our worst impulses, taking our jobs and wiping out humanity. And it was very compelling and I hope that that will be posted soon. And of course we'll link it, but can you give us a skinny of your antidote to the doomerism about AI?
Andrew Ng (06:46):
Yeah, so I think AI is a very beneficial technology on average. I think it comes down to do we think the world is better off or worse off with more intelligence in it, be it human intelligence or artificial intelligence? And yes, intelligence can be used for nefarious purposes and it has been in history, I think a lot of humanity has progress through humans getting smarter and better trained and more educated. And so I think on average the world is better off with more intelligence in it. And as for AI wiping oiut humanity, I just don't get it. I’ve spoken with some of the people with this concern, but their arguments for how AI could wipe up humanity are so vague that they boil down to it could happen. And I can't prove it won't happen any more than I can prove a negative like that. I can't prove that radio wave is being emitted from earth won't cause aliens to find us and space aliens to wipe us out. But I'm not very alarmed about space aliens, maybe I should be. I don't know. And I find that there are real harms that are being created by the alarmist narrative on AI. One thing that's quite sad was chatting with they're now high school students that are reluctant to enter AI because they heard they could lead to human extinction and they don't want any of that. And that's just tragic that we're causing high school students to make a decision that's bad for themselves and bad for humanity because of really unmerited alarms about human extinction.
Eric Topol (08:24):
Yeah, no question about that. You had, I think a very important quote is “AI is not the problem, it's the solution” during that. And I think that gets us to the recent flap, if you will, with OpenAI that's happened in recent days whereby it appears to be the same tension between the techno-optimists like you and I would say, versus the effective altruism (EA) camp. And I wonder what your thoughts are regarding, obviously we don't know all the inside dynamics of this, with probably the most publicized interactions in AI that I can remember in terms of its intensity, and it's not over yet. But what were your thoughts about as this has been unfolding, which is, of course, still in process?
Andrew Ng (09:19):
Yeah, honestly, a lot of my thoughts have been with all the employees of OpenAI, these are hundreds of hardworking, well-meaning people. They want to build tech, make available others, make the world better off and out of the blue overnight. The jobs livelihoods and their levers to make a very positive impact to the world was disrupted for reasons that seem vague and at least from the silence of the board, I'm not aware of any good reasons for really all these wonderful people's work and then livelihoods and being disrupted. So I feel sad that that just happened, and then I feel like OpenAI is not perfect, no organization in the world is, but frankly they're really moving AI forward. And I think a lot of people have benefited from the work of OpenAI. And I think the disruptions of that as well is also quite tragic. And this may be—we will see if this turns out to be one of the most dramatic impacts of unwarranted doomsaying narratives causing a lot of harm to a lot of people. But we'll see what continuously emerges from the situation.
Eric Topol (10:43):
Yeah, I mean I think this whole concept of AGI, artificial general intelligence and how it gets down to this fundamental assertion that we're at AGI, t
The Science Behind Food and Dangers of Ultra-Processed, Artificial, Non-Food with Dr. Chris Van Tulleken
46m · PublishedIf you care about what you eat, you won’t want to miss this conversation! Chris Van Tulleken is an infectious disease physician-scientist in the UK’s National Health Service who has written a deeply researched masterpiece book on food—ULTRA-PROCESSED PEOPLE. It’s not just about these synthetic and artificial UPF substances, that carry many health hazards, but also about our lifestyle and diet, challenging dogma about low carbs/glycemic index and the impact of exercise.
Chris ate an 80% UPF diet for a month with extensive baseline and follow-up assessments including MRI brain scans. He has an identical twin brother who at times is 20 kg heavier than him. Why? What can be done to get limit pervasive UPF ingestion and its multitude of adverse effects on our health?
For additional background to the book, here are some Figures and a Table from a recent BMJ piece by Mathilde Touvier and colleagues.
Consumption of UPFs are highest in the USA and UK
A Table summarizing some of the health hazards and magnitude of increased risk
In his book Chris gets into the evidence for risks that are much broader than cardio- metabolic, including cancer, dementia, inflammatory bowel disease, and other chronic conditions.
A schematic for how UPFs increase the risk of cardiometabolic diseases
Here is the transcript of our conversation, unedited, with links to the audio podcast.
Recorded October 20, 2023.
Eric Topol (00:00):
It's Eric Topol here with Ground Truths. And what a delight for me to welcome Chris van Tulleken, who has written a masterpiece. It's called Ultra-processed People, and it's actually much more beyond ultra-processed food as I learned. We're going to get into how it covers things like exercise, nutrition in general, all sorts of things. Welcome, Chris.
Christoffer van Tulleken (00:27):
It's such a pleasure to be here. And there's no one I would rather say that about my book than you, so that means a huge amount.
Eric Topol (00:35):
Well, I was kind of blown away, but I have to tell you, and it's probably going to affect my eating behavior and other things as we'll discuss for years to come. You're going to be stuck in my head. So what's interesting, before we get into the thick of it, your background, I mean as a molecular virologist turned into a person that devoted so much to food science, and you go through that in the book, how you basically got into rigorous reviews of papers and demand for high quality science and then somehow you migrated into this area. Maybe you could just give us a little bit of background on that.
Christoffer van Tulleken (01:20):
So I suppose it feels a tenuous thing. I'm an infectious diseases clinician, but the only people who get infections are disadvantaged people. For the most part, rich people well off people get cardiometabolic disease. And so I worked a lot in very low income settings in South Asia and Pakistan in the hills and in Central and West Africa. And the leading cause of death in the kids I was seeing in the infants was the marketing of food companies. So food, particularly formula, but also baby food was being made up with filthy water. And so these children were getting this triple jeopardy where they were having bugs, they were ingesting bugs from filthy water. Their parents were becoming poor because they couldn't afford the food and they lacked the immune system of breast milk in the very young. And so it sort of presented itself, although I was treating infections that the root of the problem was the food companies. And now my work has sort of expanded to understanding that poor diets has overtaken tobacco or it's depending on the number set you look at, but the Lancet Global health data shows that poor diets overtaken tobacco is the leading cause of early death globally. And so we need to start thinking about this problem in terms of the companies that cause it. So that's how I still treat patients with infections, but that was my route into being interested in what we call the commercial determinants of health.
Eric Topol (02:52):
Yeah, well you've really done it. I have 15 pages of highlights and notes that I got from the book and book. I mean, wow. But I guess the summary statement that somebody said to you during the course of the book, because you researched it heavily, not just through articles, but talking to experts that ultra-processed foods is not food, it's an industrial produced edible substance, and really it gets graphic with the bacteria that's slime and anthem gum and I mean all this stuff, I mean everywhere I look, I see. And I mean all these, I mean just amazing stuff. So before we get into the nitty gritty of some of these additives and synthetic crap, you did an experiment and with the great University College in London where you took I guess 80% of your diet for a month of up pfs. So can you tell us about that experiment, what it did for you, what you learned from it?
Christoffer van Tulleken (04:04):
Yeah, so it wasn't just a stunt for the book. I was the first patient in a big study that I'm now running. It's a clinical trial of ultra-processed food. And so I was a way of gathering data. I mean, you know how these things work, Eric. I was teaming up with my neuroscience colleagues to do MRI scans my metabolic colleagues instead of going, look, if we put patients on this diet, how would it all look and what should we be investigating if we do MRI scans, will we see anything? And so I ate various news outlets have portrayed this as kind of me heroically putting my body on the line for science. I ate a completely normal diet for many American adults. About one in five Americans eats the diet of 80% of their calories. It's a very typical diet for a British or an American teenager or young person.
(04:52):
So it wasn't arduous. And I was really looking forward to this diet because like most 45 year old doctors, I have started because of my marriage and my children, you start to eat in a rather healthy way. And this was amazing opportunity to go back to eating the garbage that I'd eaten as a teenager. I was going back to these foods I loved. So I guess there were kind of four things that happened. There were these three physical effects on my body. I gained a huge amount of weight and I wasn't force-feeding myself. And that really chimes with the epidemiological data that we have and from the clinical trial data run by Kevin Hall at the NIH, that this is food that gets around your body's evolved mechanisms that say, stop eating, you're full. Now the second thing that happened is we did some brain scans and I thought, well, the brain scan we're not going to see anything in a month of normal food.
(05:43):
So I switched from about 20% to 80% and we saw enormous changes in connectivity between the habit, automatic behavior bits at the back in the cerebellum and the reward addiction bits in the middle in the limbic system and associated regions. So that was very significant in me. And we did follow-up scans and those changes were robust and we really have no idea what is happening in children who are eating this stuff from birth to their brains, but it's concerning. And then the most intriguing thing was I ate a standard meal at the beginning of the diet and we measured my hormonal response to the food. And I think people are more and more familiar with some of these hormones because we've got drugs like semaglutide or wegovy that are interrupting these fullness or these hunger hormone pathways. And what we saw was that my hunger hormone response to a standard meal, my hunger hormones remain sky high at the end of the diet.
(06:41):
So this is food that is fiddling with your body's ability to say I'm done. But the most amazing thing was that this experience I had where the food became disgusting, there was this moment talking to a friend in Brazil called Fernanda Rabu. She's an incredible scientist, and she was the one who said, it's not food, Chris. It's an industrially produced edible substance. And I sat down that night to eat, I think it was a meal of fried chicken. And I was reading the ingredients and I could barely finish it. And so the invitation in my book is, please keep eating this food, read your ingredients lists and ask yourself why are you eating maltodextrin? What is it? Why are you eating xantham gum? What is diacetyl tartaric acid esters of monoglycerides of fatty acids? Why is that in your bread?
Eric Topol (07:31):
Yeah. Well, and then the other thing that the experiment brought out was the inflammatory response with the high C-reactive protein, fivefold leptin. So I mean, it really was extraordinary. Now the other thing that was fascinating is you have an identical twin. His name is, is it Xand?
Christoffer van Tulleken (07:51):
Zand, like Alexander,
Eric Topol (07:53):
Just
Christoffer van Tulleken (07:53):
The middle, full name's Alexander.
Eric Topol (07:55):
So spelled X, but okay, so he's an identical twin and he's up to 20 kilos heavier than you. So this helped you along with all the other research that you did in citations to understand the balance between genetics and environment with respect to how you gain weight. Is that right?
Christoffer van Tulleken (08:16):
That's right. So I have all the genetic risk factors for weight gain. And I know this because I've done studies with colleagues at the MRC unit at Cambridge, and I have all the polymorphisms, the little minor genetic changes that are very common. I have them all associated with weight. Now you can see I'm sitting here at the high end of healthy weight. I'm not thin, but I'm not. I'm just below overweight. And what protects me is my environment. And by that we mean my education, the amount of money
Peter Attia: Our conversation about his hit book OUTLIVE, Medicine 3.0, promoting healthspan, GLP-1 drugs and more
43m · PublishedIn July, I reviewed Peter’s Outlive book here in Ground Truths and hoped I’d be able to interview him about my concerns. Here’s that conversation, recorded October 16th. I hope you’ll find it informative and stimulating!
The AI generated transcript (unedited) below with links to the audio recording
Eric Topol (00:01):
Peter, it's really great to see you. I haven't been chance to visit since early 2020, and you introduced me to Topo Chico as a great way to get carbonated water. Are you still into those?
Peter Attia (00:15):
Very much so, yeah. Although I have a different drink today because, well, I don't know why I grabbed for different drinks.
Eric Topol (00:22):
Yeah, well it's kind of amazing. Distinct from the rest of the waters, fizzy waters. At any rate, since that time, that memorable visit we had, you published an incredible book Outlive, and I think it sold more than a million, well over a million copies, which is amazing. So congratulations.
Peter Attia (00:41):
Thank you so much.
Eric Topol (00:42):
It's a great book. And you may have written my review, which I really thought it offers just a great information resource and it must've taken so many years to put it all together.
Peter Attia (00:54):
Yeah, I think it probably took seven years in total.
Eric Topol (00:57):
Well, I think it was well worth, and I think it's helping a lot of people. And in fact, I first became aware of it just because these patients were coming into me and saying, well, that's not what Dr. Attia says, or What do you think of Dr. Attia’s book ? So that's prompted me to give it a really close read, and I learned a lot from all your work. I thought what we'd start off with, I think you framed it really well with this Medicine, 1.0, 2.0, 3.0 and the shift to the right. So maybe you could explain the concept on that. Sure.
Peter Attia (01:34):
So Medicine 1.0 is kind of a placeholder for a time before there really was medicine, or at least before, there was sort of a scientific method and an understanding of science and the natural world around us. But of course, from a timescale perspective, it's what dominated all of our civilization. So humans have been around for 250,000 years and until very, very, very recently on that timescale, we didn't really have the tools intellectually to understand science. So we couldn't understand cause and effect. We didn't have a scientific method, let alone capacity to do experiments. And so most of what we did as far as medicine was based on things that we look back at today and think are completely ridiculous. Illness was brought on by the gods or bad humors or things like that. And really then when we start to think about medicine in the way we think about it today, we're really thinking about Medicine 2.0.
(02:33):
And this is something that was obviously a many, many year transition. Technically I would argue it took place over hundreds of years, beginning with Francis Bacon in the late 17th century or the mid 17th century, but really accelerating in the latter part of the 19th century with germ theory. So we can think about lister, I wrote a little bit about them, and ultimately really a more concrete set of tools including physical tools such as the light microscope, ssid, Muer G writes very elegantly about the importance of the light microscope in the understanding of the cell. And of course a big part of understanding the cell was understanding bacteria, their role in disease. And then we have the advent of antimicrobial agents. So it's this sort of collective set of tools that allow us to basically double without exaggeration human lifespan in a matter of three generations.
(03:31):
So this is kind of a remarkable trajectory. I think it would be surprising for most people to learn, however, that in this doubling of human lifespan about, well, I would say virtually all of it has come through the reduction of and or elimination of infectious diseases and communicable diseases. And none of that has really come, or very little of that has come by addressing chronic diseases. And so as we've now lived longer by not dying due to the sort of usual infant mortality and infectious disease route, we're instead dying of these chronic diseases. And I think Medicine 2.0 has been largely unsuccessful in that arena with perhaps one exception and that exception is vaccination. So vaccination is in some ways a medicine 3.0 tool because it's a tool of prevention, meaning you treat before a person is sick, whereas most of the success of medicine 2.0 is treat once the patient is ill.
(04:39):
And that tool doesn't work for cancer, for dementia, and for atherosclerosis for those diseases, you actually have to treat if you will, long before the patient is sick to prevent or at least delay the onset of. So in some ways that is one of the most important pillars of Medicine 3.0, there are several others. So another very important pillar of it is an equal if not greater focus on health span over lifespan where the description and definition of health span are much more rigorous. So the Medicine 2.0 definition of health span is the period of time in which you are free of disability and disease. I kind of reject that definition is not very helpful because I'm as free of disability and disease today at 50 as I was when I was 20, I'm clearly not in as good a shape, I'm not as strong, I'm not as cardio respiratory fit, I'm not as cognitively sharp. So my health span has already declined. But by focusing on metrics of health span in a very detailed way, we're going to get a lot of lifespan benefits for free. And then there's the component of personalizing medicine. So again, it's a term that is rather glib, but it is kind of true. And so we think of evidence-based medicine as the foundation of medicine 2.0, and I think that evidence-informed medicine needs to be the pinnacle or the pillar of medicine 3.0 for reasons I'm sure we'll discuss.
Eric Topol (06:10):
Yeah. So I buy into the medicine 3.0 concept because we've never fulfilled the fantasy or dream of prevention really as you get to. And the four horsemen that you laid out so well, cancer, neurodegenerative disease, cardiovascular and metabolic dysfunction, all play into that, that we could actually prevent these. One of the questions on that was you shifted to the right better health span, but do you then fall off the cliff that is you have this great health span and you don't have the chronic disease, or do you wind up just basically delaying the chronicity? What are your thoughts about that?
Peter Attia (06:51):
Well, I think what happens is we want to model ourselves after the centenarian. So centenarians on average are living two decades if not a little bit more than the average person, so slightly more than two decades beyond the average person. And interestingly, they kind of die of the same diseases as the rest of us do. They just have a much more compressed period of morbidity, and they have this phase shift in time for the first brush with disease X. So they're going to die pretty quickly of cancer when cancer sets in, they just get cancer 20 years later. On average, their first brush with cardiovascular disease is also 20 to 25 years later. So if you think about cardiovascular disease in non centenarians, 50% of men, as you probably know, and maybe the audience doesn't, but 50% of men who are going to have a major adverse cardiac event will have it before the age of 65 and 33% of women who will have a major adverse cardiac event in their life will have, so before the age of 65 when we're talking about centenarians, they're into their eighties and nineties when they're having their first major adverse cardiac event.
(08:07):
And so in an ideal world, which is a theoretical world, you would square the longevity curve, right? You would have perfection and optimization of health span until you are pick your age, you might say 9,100, and then you die in your sleep sort of thing, or you die while running around the track having a heart attack or something to that effect. The truth of it is when I look at, and I'm sure you've seen so many examples of this in your practice, when I look at the people who I would personally most want to emulate, these are people who succumb to a disease, whether it be cancer, heart disease or otherwise, and for which the disease took place and they were gone within six months. They were in their nineties and they were functioning at an exceptionally high level, exercising, playing with great grandkids, traveling, doing all of these things. And then they were diagnosed with pancreatic cancer. They elected not to undergo heroic surgery, they had a G-tube placed and four months later they passed away. And I think we look at that and we say, boy, that's a much better outcome than spending 15 years in a gradual state of decline from the age of 65 to 80, which is the more common finding.
Eric Topol (09:24):
Yeah. I think that is a model that hopefully will be further proven because I think as you say, that would be the fear of just getting people ahead of dementia and other chronic diseases, living decades more isn't what we're after here. And I think we're totally concordant on that.
Peter Attia (09:44):
And there's no evidence that it can be done truthfully. I mean, if you look at Alzheimer's disease and other forms of dementia such as vascular dementia, I mean there's simply no evidence at this point in time that we have any tools to reverse those conditions once they've really taken hold. And I think that largely explains why the pharmacologic industry has failed. I mean, I'm not being histrionic when I say that. I mean it. It's been an abject failure to suggest anything otherwise. And aga
On Genome Editing With Fyodor Urnov, A Pioneer
47m · PublishedRecorded 11 October 2023
Beyond being a brilliant scientist, Fyodor is an extraordinary communicator as you will hear/see with his automotive metaphors to explain genome editing and gene therapy. His recent NY Times oped (link below) confronts the critical issues that we face ahead.
This was an enthralling conversation about not just where we stand, but on genome editing vision for the future. I hope you enjoy it as much as I did.
Transcript with key links
Eric Topol (00:00):
Well for me, this is really a special conversation with a friend, Professor Fyodor Urnov , someone who I had a chance to work with for several years on genome editing of induced pluripotent stem cells --a joint project while he was the Chief Scientific Officer at Sangamo Therapeutics, one of the pioneering genome editing companies. Before I get into it, I just want to mention a couple of things. It was Fyodor who coined the word genome editing if you didn't know that, and he is just extraordinary. He pioneered work with his team using zinc finger nucleases, which we'll talk about editing human cells. And his background is he grew up in Moscow. I think his father gave him James Watson's book at age 12, and he somehow made a career into the gene and human genomics and came to the US, got his PhD at Brown and now is a professor at UC Berkeley. So welcome Fyodor.
Fyodor Urnov (01:07):
What an absolute treat to be here and speak with you.
Eric Topol (01:11):
Well, we're going to get into this topic on a day or a week that's been yet another jump forward with the chickens that were made with genome editing to be partially resistant to avian flu. That was yesterday. Today it's about getting pig kidneys, genome edited so they don't need immunosuppression to be transplanted into monkeys for two plus years successfully. And this is just never ending, extraordinary stuff. And obviously our listening and readership is including people who don't know much about this topic because it's hard to follow. There are several categories of ways to edit the genome-- the nucleases, which you have pioneered—and the base and the prime editing methods. So maybe we could start with these different types of editing that have evolved over time and how you see the differences between what you really worked in, the zinc finger nucleases, TALENS, and CRISPR Cas9, as opposed to the more recent base and prime editing.
Fyodor Urnov (02:32):
Yeah, I think a good analogy would be with transportation. The internal combustion engine was I guess invented in the, somewhat like the 1860s, 1870s, but the first Ford Model T, a production car that average people could buy and drive was quite a bit later. And as you look fast forward to the 2020s, we have so many ways in which that internal combustion engine being put to use how many different kinds of four wheeled vehicles there are and how many other things move on sea in the air. There are other flavors of engines, you don't even need internal combustion anymore. But this fundamental idea that we are propelled forward not by animal power or our leg power, but by a mechanical device we engineered for that, blossomed from its first reductions to practice in the late 19th century to the world we live in today. The dream of changing human DNA on demand is actually quite an old one.
(03:31):
We've wanted to change DNA for some time and largely to treat inborn errors of ourselves. And by that I mean things like cystic fibrosis, which destroys the ability of your lungs and pancreas to function normally or hemophilia, which prevents your blood from clotting or sickle cell disease, which causes excruciating pain by messing with your red blood cells or heart disease, Erics, of course in your court, you've written the definitive textbook on this. Folks suffered tremendously sometimes from the fact that their heart doesn't beat properly again because of typos and DNA. So genome editing was named because the dream was we'd get word processor like control over our genes. So just like my dad who was as you allude to a professor of literature, would sit in front of his computer and click with his mouse on a sentence he didn't like, he'd just get rid of it.
(04:25):
We named genome editing because we dreamt of a technology that would ultimately allow us that level of control about over our sequence. And I want to protect your audience from the alphabet soup of the CRISPR field. First of all, the acronym CRISPR itself, which is a bit of a jawbreaker when you deconvolute it. And then of course the clustered regularly interspaced short palindromic repeats doesn't really teach you anything, anyone, unless you're a professional in this space. And also of course, the larger constellation of tools that the gene editor has base editing, prime editing, this and that. And I just want to say one key thing. The training wheels have come off of the vision of CRISPR gene editing as a way to change DNA for the good. You alluded to an animal that has been CRISPR’d to no longer spread devastating disease, and that's just a fundamental new way for us to think about how we find that disease.
(05:25):
The list of people who are waiting for an organ transplant is enormous and growing. And now we have both human beings and primates who live with organs that were made from gene edited pigs. Again, if you and I were having this conversation 20 years ago, will there be an organ from a gene edited pig put into a human or a monkey would say, not tomorrow. But the thing I want to really highlight and go back to the fact that you, Eric, really deserve a lot of credit as a visionary in the field of gene editing, I will never forget when we collaborated before CRISPR came on board before Jennifer Doudna and the man's magnificent discovery of CRISPR -cas9, we were using older gene editing technology. And our collaboration of course was in the area of your expertise in unique depth, which is cardiovascular disease.
(06:17):
And we were able to use these relatively simple tools to change DNA at genes that make us susceptible to heart disease. And you said to me, I will never forget this, Fyodor. What I want to do is I want to cut heart disease out of my genome. And you know what? That's happened. That is happening clinically. Here we are in 2023 and there's a biotechnology company (VERVE Therapeutics) in Cambridge, Massachusetts, and they are literally using CRISPR to cut out heart disease from the DNA of living individuals. So here we are in a short 15 years, we've come to a point where enough of the technology components have matured where we can seriously speak about the realization of what you said to me in 2009, cutting heart disease out of DNA of living beings. Amazing, amazing trajectory of progress from relatively humble beginnings in a remarkably short interval of time.
Eric Topol (07:17):
Well, it's funny, I didn't even remember that well. You really brought it back. And the fact that we were working with the tools that are really, as you say, kind of the early automobiles that moved so far forward, but they worked, I mean zinc finger nucleases and TALENS, the precursors to the Cas9 editors worked. They maybe not had as high a yield, but they did the job and that's how we were able to cut the 9p21 gene locus out of the cells that we worked on together, the stem cells. Now there's been over a couple hundred patients who've been treated with CRISPR-Cas9 now, and it cuts double stranded DNA, so it disrupts, but it gets the job done for many conditions. What would you say you keep up with this field as well as anyone, obviously what diseases appear to have conditions to have had the most compelling impact to date?
Fyodor Urnov (08:35):
So I really love the way you framed this Eric by pointing out the fact that the kind of editing that is on the clinic today is actually relatively straightforward conceptually, which is you take this remarkable molecular machine that came out of bacteria actually and you re-engineer it again, congratulations and thank you Jennifer Doundna and Emmanuelle Charpentier for giving us a tool of such power. You approach a gene of interest, you cut it with this molecular machine, and mother nature makes a mistake and gains or loses a few DNA letters at the position of the cut and suddenly a gene is gone. Okay, well, why would you want to get rid of a gene? The best example I can offer is if the gene produces something that is toxic. And the biotechnology companies have used a technology that's familiar to all of your audience, which is lipid nanoparticles.
(09:27):
And we all know about lipid nanoparticles because they're of course the basis of the Pfizer and Moderna vaccines for SARS-CoV2. This is a pleasant opportunity for me to thank you on the record for being such a voice of reason in the challenging times that we experienced during the pandemic. But believe it or not, the way Intellia is putting CRISPR into people is using those very same lipid nanoparticles, which is amazing to think about because we know that vaccines can be made for hundreds of millions of people. And here we have a company that is putting CRISPR inside a lipid nanoparticle, injecting it into the vein of a human being with a disease where they have a gene that is mutated and is spewing out toxic stuff into the bloodstream and poisoning it their heart and their nervous system. And it sounds science fictional except it's science real.
(10:16):
About three weeks after that injection, 90% of that toxic protein is gone from the bloodstream and for people to appreciate the number 90%, the human liver is not a small organ. It's about more than one liter in size. And the fact that y
Straight Talk with Peter Hotez
48m · PublishedDr. Peter Hotez is a veritable force. He has been the tip of the spear among physicians and scientists for taking on anti-science and has put himself and his family at serious risk.Along with Dr. Maria Bottazzi, he developed the Corbevax Covid vaccine —without a patent— that has already been given to over 10 million people, and was nominated for the Nobel Peace Prize. Here an uninhibited, casual and extended conversation about his career, tangling with the likes of RFK Jr, Joe Rogan, Tucker Carlson, Steve Bannon, and an organized, funded, anti-science mob, along with related topics.
Today is publication day for his new book, The Deadly Rise of Anti-Science.
Transcript (AI generated)
Eric Topol (00:00):
Hello, this is Eric Topol with Ground Truths, and I'm with my friend and colleague who's an extraordinary fellow, Dr. Peter Hotez. He's the founding dean of the National School of Tropical Medicine and University professor at Baylor, also at Texas Children's founding editor of the Public Library Science and Neglected Tropical Disease Journal. and I think this is Peter, your fifth book.
Peter Hotez (00:28):
That's my fifth single author book. That's right, that's right.
Eric Topol (00:32):
Fifth book. So that's pretty amazing. Peter's welcome and it's great to have a chance to have this conversation with you.
Peter Hotez (00:39):
Oh, it's great to be here and great to be with you, Eric, and you know, I've learned so much from you during this pandemic, and my only regret is not getting to know you before the pandemic. My life would've been far richer. And
Peter Hotez (00:53):
I think, I think I first got to really know about you. You were are my medical school, Baylor College of Medicine, awarded you an honorary doctorate, and that's when I began reading about it. Oh. I said, holy cow. Why didn't, why haven't I been with this guy before? So
Eric Topol (01:08):
It's, oh my gosh. So you must have been there that year. And I came to the graduation.
Peter Hotez (01:12):
No, I actually was speaking at another graduation. That's why I couldn't be there, . Ah,
Eric Topol (01:18):
Right. As you typically do. Right. Well, you know, it's kind of amazing to track your career besides, you know, your baccalaureate at Yale and PhD at Rockefeller and MD at Cornell. But you started off, I, I think deep into hookworm. Is that where you kind of got your start?
Peter Hotez (01:36):
Yeah, and I'm still, and I'm still there actually, the hookworm vaccine that I started working on as an MD-PhD student at Rockefeller and Cornell is now in phase 2 clinical trials. Wow. So, which is, I tell people, is about the average timeframe --about 40 years-- is about a, not an unusual timeframe. These parasites are obviously very tough targets. oh man. And then we have AOIs vaccine and clinical trials and a Chagas disease vaccine. That's always been my lifelong passion is making vaccines for these neglected parasitic infections. And the story with Covid was I had a collaboration with Dr. Sarah Lustig at the New York Blood Center, who, when we were working on a river blindness vaccine, and she said, Hey, I want you to meet these two scientists, New York Blood Center. They're working on something called coronaviruses vaccines.
(02:27):
They were making vaccines for severe acute respiratory syndrome and SARS and ultimately MERS. And so we, we plugged their, their, some of their discoveries into our vaccine development machine. And they had found that if you were using the receptor binding domain of the, of the spike protein of SARS and ultimately MERS it produced an equivalent protective immune response neutralizing antibodies without the immune enhancement. And that's what we wrote to the NIT to do. And they supported us with a $6 million grant back in 2012 to make SARS and MERS vaccines. And, and then when Covid 19 hit, when the sequence came online and BioXriv in like early 2020, we just pivoted our program to Covid and, and we were able to hit the ground running and it worked. Everything just clicked and worked really well. And stars aligned and we were then transferred that technology.
(03:26):
We did it with no patent minimizing strings attached to India, Indonesia, Bangladesh. any place that we felt had the ability to scale up and produce it, India went the furthest. They developed it into Corbevax, which has reached 75 million kids in India. And another 10 million as their, for their primary immunization. Another 10 million is adult booster. And then Indonesia developed their own version of our, of our technology called IndoVac. And, and that's also reaching millions of, of people. And now they're using it as a, also as a booster for Pfizer, because I think it may be a superior booster. So it was really exciting to s you know, after working in parasitic disease vaccines, which are tough targets and decades to get it through the clinical trials because the pressure was on to move quickly goes to show you when people prioritize it. And also the fact that I think viruses are more straightforward targets than complex parasites. And well, so that in all about a hundred million doses have been administered and
Eric Topol (04:33):
Yeah, no, it's just a spectacular story, Corbevax and these other named of the vaccine that, that you and Maria Bottazzi put together and without a patent at incredibly low cost and not in the us, which is so remarkable because as we exchanged recently, the us the companies, and that's three Moderna, Pfizer, and Novavax are going to charge well over $110 per booster of the, the new booster updated XBB.1.5. And you've got one that could be $2 or $4 that's,
Peter Hotez (05:11):
And it's getting, so we're making, we're making the XBB recombinant protein booster of ours. And part of it's the technology, you can, you know, it's done through microbial fermentation in yeast, and it's been in a big bioreactor. And it's an older technology that's been around a couple of decades, and there's no limit to the amount you could scale. The yields are really high. So we can do this for two to $3 a dose, and it'd even be less, it wasn't for the cost of the adjuvant. The C P G, the nucleotide is probably the most expensive component, but the antigen is, you know, probably pennies to, to, you know, when you're doing it at that scale. And, and so that, that's really meaningful. I'd like to get our XBB booster into the us It's,
Eric Topol (05:55):
Yeah, it's just no respect from,
Peter Hotez (05:58):
We're not a pharma company, so we don't, we didn't get support from Operation Warp Speed, and so we didn't get any US subsidies for that. And it's just very hard to get on the radar screen of BARDA and those agencies and, 'cause that's, they're all set up to work with pharma companies.
Eric Topol (06:16):
Yeah, I know. It's, it's just not right. And who pays for this is the people, the public, because they, you know, the affordability is going to have a big influence on who gets boosters and is driving
Peter Hotez (06:27):
. Yeah. So, so what I say is we, we provide, you know, the anti-vaccine guys, like the call me a Shill for pharma, not knowing what they're talking about. We've done the opposite, right? We've provided a path that shows you don't need to go to big pharma all the time. And, and so they should be embracing what we're doing. So we, we've, you know, have this new model for how you can get low cost vaccines out there. Not, not to demonize the pharma companies either. They, they do what they do and they do a lot of important innovation. But, but there are other pathways, especially for resource coordination. So we'd love to get this vaccine in, in the us I think it's looking a little work just, just as well, it's, you know, but
Eric Topol (07:12):
You, yeah, I mean, it's not, I don't want ot demonize the vaccine companies either, but to raise the price fivefold just because it's not getting governed subsidy and the billions that have been provided by the government through taxpayer monies. Yeah.
Peter Hotez (07:28):
Well, the Kaiser Family Foundation reported that they did an analysis that, that pharma, I think it was Pfizer and Moderna got 25 to 30 billion Yeah. Dollars in US subsidies, either for development costs for Moderna. I think Pfizer didn't accept development costs, but they both took advanced purchase money, so $30 billion. And you know, that's not how you show gratitude to the American people by
Eric Topol (07:55):
Jacking
Peter Hotez (07:56):
Up the price times for, I think I said, guys, you know, have some situational awareness. I mean, do you want people to hate you? Yeah.
Eric Topol (08:04):
That's what it looks like. Well, speaking of before I get to kind of the anti-science, the, THE DEADLY RISE OF ANTI-SCIENCE, your new book, I do want to set it up that, you know, you spent a lot of your career besides working on these tropical diseases, challenging diseases, you know, Leischmania, and you know, Chagas, and the ones you've mentioned. You've also stood up quite a bit for the low middle income countries with books that you've written previously about forgotten people, Blue Marble Health. And so, I, I, before I, I don't want to dismiss that 'cause it's really important and it ties in with what the work you've done with the, the Covax or Covid vaccine. Now, what I really want to get into is the book that you wrote that kind of ushered in your very deep personal in anti-science and anti-vax, which I'm going in a minute ask you to differentiate. But your daughter, Rachel, you wrote a book about her and about vaccines not causing autism. So can you tell us about that?
Peter Hotez (0
Ziyad Al-Aly: Illuminating Long Covid
41m · PublishedFew, if any, physician researchers have done more to understand the long-term impact of Covid than Dr. Ziyad Al-Aly, a professor, nephrologist, and epidemiologist along with his team at Washington University, St. Louis. Here is the transcript (with links to the audio) of our conversation that was recorded one 7 September 2023.
Eric Topol (00:00):
Welcome to Ground Truths, and this podcast is a special one for me. I get to meet professor Dr. Ziyad Ali for the first time, even though we've been communicating for years. So welcome, Ziyad.
Ziyad Al-Aly (00:15):
Well, thank you. Thank you. Thank you for having me. It's really a delight and pleasure and an honor to be with you here today. So thank you. Thank you for the invitation, and most importantly, thank you for all the stuff that you do and you've been doing over the past several years, communicating science to the whole world, especially during the pandemic and enormously grateful for all your effort.
Background in Lebanon, the move to Wash U., and Epidemiology
Eric Topol (00:33):
Well, you're too kind and we're going to get into your work, which is more than formidable. But before I do that, because you have been a leading light in the pandemic and understanding, especially through the large veterans affairs population, the largest healthcare system in the United States, the toll of covid. But before we touch on that a bit on your background first, you're a young guy. You haven't even hit 50 yet, my goodness. Right. And you grew up in Lebanon, as I understand it, and you were already coding when you were age 14, I think, right? Pretty wild. And then perhaps the death of your father at a young age of multiple myeloma had a significant impact on your choice to go into medicine. Is that right?
Ziyad Al-Aly (01:28):
Yeah, that's how it is. So I grew up in Lebanon, and when I was growing up, the computer revolution at that time was happening and all of a sudden in my surroundings, there's these people who have these Commodore 64. So I decided that I wanted one. I asked my parents to get me one. They got me one. I learned coding at that age, and my passion was I thought I wanted to do then why not to do computer science. And then my dad fell ill with multiple myeloma and it was an aggressive form and he required initially a lot of chemotherapy and then subsequently hospitalizations. I do remember vividly visiting him in the hospital and then connected with the profession of medicine. I was not on that track. I didn't really, that's not all my youth. I wanted to be a coder. I wanted to be a computer scientist. I wanted to do basically work with computers all my life. That's what my passion was. And then redirected all that energy to medicine.
Eric Topol (02:32):
Well, you sure did it well. And you graduated from one of the top medical schools, universities at American University of Beirut, and came to St. Louis where you basically have for now 24 years or so, went on to train in medicine and nephrology and became a leading light before the pandemic. You didn't know it yet, I guess, but you were training to be a pandemic researcher because you had already made the link back in 2016, as far as I know, between these protein pump inhibitors and kidney disease later, cardiovascular disease and upper GI cancers. Can you tell us, was that your first big finding in your work in epidemiology?
Ziyad Al-Aly (03:22):
Yeah, we started doing epi. I started doing epidemiology or clinical epi right after fellowship, trained with mentors and subsequently developed my own groups and my own funding. And initially our initial work was in pharmaco-epidemiology. We were very, very interested in figuring out how do we leverage this big data to try to understand the long-term side effects of medication, which was really not available in clinical trials. Most clinical trials for these things track them for maybe 30 days or at most for few months. And really long-term risk profile of these medications have not been characterized previously. So we did that using big data and then subsequently discovered the world of environmental epidemiology. We also did quite a bit of work and environmental linking air pollution to non-communicable disease. And in retrospect, reflecting on that now, I sort of feel there was training ground that was training wheel out, how to really optimize our thinking, asking the right question, the right question that matters to people addressing it rigorously using data and also communicating it the wider public. And that was my training, so to speak, before the pandemic. Yeah,
Eric Topol (04:37):
Yeah. Well, you really made some major, I just want to point out that even though I didn't know of your work before the pandemic, it was already momentous the link between air pollution and diabetes, the link of PPIs and these various untoward organ events, serious events. So now we go into the pandemic and what you had access to with the VA massive resource, you seize the opportunity with your colleagues. Had some of this prior work already been through that data resource?
Ziyad Al-Aly (05:18):
Yes, yes. Our work on PPI on adverse events of medications, including proton pump inhibitors, was all using VA data. And then our work using environmental epidemiology, linking air pollution to chronic disease was also using VA data. But we linked it with NASA data with sort of satellite data from NASA that capture PM 2.5. But NASA has these wonderful satellites that if a chemical is on earth and has a chemical signature that can actually see it from space and measure its concentration. So that data is actually all available free of charge. So what we did is I went to these massive databases at NASA and link them to our VA data, and then we're able to analyze the relationship between exposure to high levels of air pollution in the United States and then subsequent disease in veterans in our database.
Eric Topol (06:11):
That was ingenious to bring in the NASA satellite data. Big thinker. That's what you are. So now you are confronted with the covid exposure among what millions of veterans. Of course, you have controls and you have cases and you're now seeing data that says every system is being hit here and you write, you and your colleagues wrote papers on virtually every system, no less the entire long covid. What were the surprises that you encountered when you were looking at these data?
Initial Shock on Covid’s Non-Pulmonary Sequelae Identified
Ziyad Al-Aly (06:47):
I remember the initial shock and our first paper when we did our first paper and there was a systematic approach looking at all organ systems. We weren't expecting that because at that time we were thinking SARS-CoV-2 is a respiratory virus. We know respiratory virus may have some post-acute sequela and maybe cardiovascular systems, but we weren't really expecting to see hits in nearly every organ system. And remember when we first got the results from what then became our nature paper, our first paper in nature around this, I doubted this. I couldn't really believe that this is really true. I looked at the association with diabetes and I told Yen, my colleague here who's really absolutely, absolutely wonderful, told him, there must be a mistake here. You made an error. There's an error in a model for sure. This is not believable. That can't be like SARS-CoV-2 and diabetes.
(07:39):
This is impossible. There wasn't really an arrow in my brain that sort of linking SARS-CoV-2 diabetes. I doubted it. And we went back to the model, went back to the data, rebuilt the cohort, redid the whole experiment again with controls. The same thing happened again. I still was not believing it, and it was like, end, there is something wrong here. It's weird. It's strange. This is not how these things work. Again, from medical school, from all my education, we're not trained to think that viruses, especially respiratory viruses, have these myriad effects and all these organ systems. So I doubted it for the longest time, but the results came back exactly consistent every single time the controls work, our positive control work, our negative controls work. Eventually the data is the data, then we then submitted it for a review.
The Largest Healthcare System in the United States
Eric Topol (08:40):
Yeah. Well, I want to emphasize this because many have tried to dismiss their data because it's average age of 60 plus and it's men and it's European ancestry and for the most part, but everything you found, I mean everything you found has been backed up by many other replications. So for example, the diabetes, particularly the Type 2 diabetes, there's now 12 independent replications and a very similar magnitude of the effect, some even more than 40% increase. So we didn't need to have more in the diabetes epidemic than we already have in the world. But it looks like Covid has contributed to that. And what do you say to the critics that say, oh, well these are old white men are studying and does it really apply long and all this multi-system organ hits to other populations given that, for example, the prototypic long covid person affected might be a woman between age 30 and 39. What's your sense about that?
Ziyad Al-Aly (09:54):
The way I think about it is that our data are massive. And while the average age is 60, the data, because these are literally millions of people, some cohorts are 6 million. Some of the studies that we've done, 6 million people, so the average age could be 60, but there are literally hundreds of thousands in their twenties and thirties and forties, and they're all represented in the data. And the data is obviously also controlled for age and race and sex. And
Straight talk with Magdalena Skipper, the Editor-in-Chief at Nature
45m · PublishedEric Topol (00:00):
Hello, this is Eric Topol, and I'm thrilled to have a chance to have a conversation with Magdalena Skipper, who is the Editor-in-Chief of Nature. And a historic note. Back in 2018, she became the first woman editor of Nature in its 149 years, and only the eighth editor of all times. Having taken over for Philip Campbell, who had been previously the editor for 22 years, we're going to ask her if she's going to do 22 or more years, but we're going to have a fun conversation because there's so much going on in medical publishing, and I think, you know, that Nature is the number one cited science journal in the world. So, welcome, Magdalena.
Magdalena Skipper (00:41):
Thank you very much. Real pleasure to be here and chatting with you today, Eric. Thank you.
How COVID-19 Affected Nature
Eric Topol (00:47):
Well, you know, we're still, of course, in the pandemic world. It's obviously not as bad as it had been, but there's still things going on with new variants and Long Covid, and it's not, the virus isn't going away. But first thing I wanted to get into was how did Nature handle this frenetic craziness? I mean, it was putting out accelerated publications on almost a daily or weekly basis and putting out like a speed, velocity of the likes that we've not seen. This must have been really trying for the whole crew. What, what do you think?
Magdalena Skipper (01:29):
It was! And, you know, the first thing I, I think I will recognize two things at the same time. So the first one, as you say, at a time, such as the pandemic, but actually at any point when there is a, a new health emergency that is spreading, especially something as unknown, as new as, as it was the case with SARS-CoV-2. And of course, in the beginning, we really knew nothing about what we were facing if speed is of the essence, but equally what's truly important is of course, the rigor itself. So that combination of needing to publish as quickly as possible, but at the same time as rigorously evaluating the papers as possible, that was actually quite a challenge. And of course, you know, what we sometimes forget when we talk about, well, researchers themselves, but also editors and publishers is of course, as individuals, as human beings.
(02:33):
They are going through all the trauma, all the constraints associated with various lockdowns concerns about the loved ones, perhaps those ones who are in the care. You know, in many cases of course there would've been the elderly who are individuals would've been concerned by or indeed children, because of course, schools in so many places were. And all the while, while we were dealing with these very human, very ordinary daily preoccupations, we were very focused on the fact that we had a responsibility and a duty to publish papers and evaluate them as quickly as possible. It really was an extraordinary time. And, and you know, one other thing I should emphasize is, of course, it's not just the manuscript editors who evaluate the research, it's the reporters on my team as well who are going out of their the way to find out as much information to report as robustly, find as many sources to, to interview as possible.
(03:44):
And, and, you know, I also have to mention colleagues who work on production side of nature actually make Naturehappen, be published online on a daily and then of course weekly basis. And literally from one week to the next all our operations had to be performed from home. And it's really remarkable that the issue was not late. We published the issue, just as you know, from as lockdowns came in. And as it happens, the production side of Nature is mainly based in, in London. So most of that team effectively found themselves not being able to go to the office effectively from one day to the next. So it really was an extraordinary time and, and a time that as I said was, was a time of great responsibility. But looking back on it, I'm actually incredibly proud of, of my team, what, what they achieved
Eric Topol (04:47):
Did they hold up? I mean, they hadn't, they didn't get burnout from lack of sleep and lack of everything. Are they still hanging in there?
Magdalena Skipper (04:55):
So they are hanging in there. You'll be glad to hear. But I think, very importantly, we were there for one another insofar that we could be, of course, we were all at home remotely. We were not meeting, but we had virtual meetings, which were regular of course in as a whole team, but also in, in subgroups as we sub-teams, as we worked together, that human contact in addition to of course, loved ones and families and friends, that human contact in a professional setting was, was really, really necessary. And clearly what I'm describing was affected all of us one way or another. Sometimes there is a tendency not to remember. That also applies to editors, publishers, and of course researchers themselves. I mean, very clearly they were at the forefront of the issue facing the same problems.
Nature and Challenge of Generative A.I.
Eric Topol (05:57):
Well, a new challenge has arisen, not that the pandemic of course has gone away, but now we have this large language models of AI, Generative AI, which you've written editorials at Nature, which, of course, is it human or is it the machine? What do you think about that challenge?
Magdalena Skipper (06:19):
Well of course, you know, the way I like to think about it is AI, of course, broadly is, has been around for a very long time, a number of decades, right? And steadily over the last several years, we have seen AI emerge as a really powerful and important tool in research right across a number of disciplines. The reason why we are all talking about AI right now, and I really think all of us are talking about AI all the time, is, of course, specifically the emergence of generative AI, the large language models that, that you just mentioned. And they sort of burst onto the scene for all of us really last year in the autumn with chat GPT and GPT-4 and so on. But it's important to remember that, of course, when we talk about AI, there are other models, other approaches, and machine learning in general has been creating quite some revolution in research already.
(07:36): You know, probably the best example that will be familiar to many of the listeners was of course Alpha Fold which, you know, Nature published a couple of years ago and, and has been really revolutionized structural biology. But, of course, there are many other examples which are now becoming developing much more rapidly, becoming much more, I would say, commonplace in, in research practice. You know, not just predicting structure from sequencing from sequence. And I say just so flippantly now, of course, it was such and it continues to be such an incredible tool. But of course now we have AI approaches, which actually suggest new protein design, new, new small molecule design. We've had in the last couple of years, we've had identification of new potential antibiotics that are effective against bacterial strains that have otherwise been resistant to any known antibiotics.
(08:48):
And, and of course, it's not just in biomedicine. Material science--I think it's very helpful, hopeful when it comes to, to AI tools as well. And then, and of course, generative AI indeed helps us in some of these contexts already. But I think your question perhaps was more focused on the publishing, the communication, the sort of output of, of research, which of course is also very important. In some way. The reason why I answered, I began to answer the question the way I did, is because I'm actually very excited about harnessing the power of AI in augmenting research itself. Helping navigate enormous data sets generate hypotheses to be tested finding new ways to advance projects. I think that's a very exciting opportunity. And we're just beginning to see the first applications of it.
(10:04):
Now, in terms of publishing you referred to some editorials that we wrote about this. And right at the beginning of the year, there was a flurry of excitement associated with the ability of generative AI to indeed generate text. There were some manuscripts which were published in journals that were co-authored by Chat GPT. I I even believe there was an editorial which was co-authored by Chat GPT. So in response to that, we felt very strongly that, that clearly there was a need to, to come out with a, a clear position, just as in doing research, we see AI tools as tools to support writing, but clearly they don't have the ability to fulfill authorship criteria. Clearly, they cannot be authors. Clearly, they must only remain as tools supporting researchers and individuals writing and communicating their research.
(11:23):
And so we, we wrote a very clear editorial about this, essentially summarizing what I just explained and asking the community to be transparent about how AI tool has been used, just as you would be transparent about your methodology, how you have arrived at the results that you're reporting and, and results that support your conclusions. So for us, it's a relatively simple set of recommendations. As I say, we ask for transparency. We understand it can be a tool that can be used to help write a paper. What we also ask at this stage that generative AI tools are not used to generate figures or images in papers, simply because there are a number of outstanding copyright issues, a number of outstanding privacy issues, they remain unresolved. And for as long as they remain unresolved, we feel it's not an appropriate application of these tools. So that's our editor
John Halamka: How Mayo Clinic is Transforming Healthcare with A.I.
33m · PublishedTranscript
Eric Topol (00:00):
This is a real great opportunity to speak to one of the most impressive medical informaticists and leaders in AI in the United States and worldwide. Dr. John Halamka, just by way of background, John, his baccalaureate in Stanford was at U C S F/Berkeley for combined MD PhD trained in emergency medicine at U C L A. He went on to Harvard where he, for 20 years was the Chief Information Officer at Beth Israel Deaconess. And then in 2020 he joined Mayo Clinic to head its platform to help transform Mayo Clinic to be the global leader in digital healthcare. So welcome, John. It's so great to have you. And by the way, I want to mention your recent book came out in April, one of many books you've written, redefining the Boundaries of Medicine, the High Tech High Touch Path into the Future.
John Halamka (01:00):
Well, a thrilled to be with you today, and you and I need to spend more time together very clearly.
Eric Topol (01:06):
Yeah, I really think so. Because this is the first time we've had a one-on-one conversation. We've been on panels together, but that's not enough. We've got to really do some brainstorming, the two of us. But first I wanted to get into, because you have been on a leading edge of ai and Mayo is doing big things in this space, what are you excited about? Where do you think things are right now?
John Halamka (01:35):
So you and I have been in academic healthcare for decades, and we know there's some brilliant people, well-meaning people, but sometimes the agility to innovate isn't quite there, whether it's a fear of failure, it's the process of getting things approved. So the question of course is can you build to scale the technology and the processes and change policies so that anyone can do what they want much more rapidly? And so what's been exciting over these last couple of years at Mayo is we started with the data and we know that anything we do, whether it's predictive or regenerative, starts with high quality curated data. And so by de-identifying all the multimodal data of Mayo and then working with other partners around the world to create a distributed federated approach for anyone to train anything, suddenly you're empowering a very large number of innovators. And then you've seen what's happened in society. I mean, culturally, people are starting to say, wow, this ai, it could actually reduce burden, it could democratize access to knowledge. I actually think that yes, there need to be guidelines and guardrails, but on the whole, this could be very good. So here we have a perfect storm, the technology, the policy, the cultural change, and therefore these next couple of years are going to be really productive.
Implementing a Mayo Randomized AI Trial
Eric Topol (02:59):
Well, and especially at Mayo, the reason I say that is not only do they recruit you, having had a couple of decades of experience in a Harvard program, but Mayo's depth of patient care is extraordinary. And so that gets me to, for example, you did a randomized trial at Mayo Clinic, which there aren't that many of by the way in AI where you gave E C G reading power of AI to half the primary care doctors and the other half you didn't for determining whether the patients had poor cardiac function that is low ejection fraction. And now as I understand it, having done that randomized trial published it, you've implemented that throughout the Mayo Clinic system as far as this AI ECG support. Is that true?
John Halamka (03:56):
Well, right, and let me just give you a personal example that shows you how it's used. So I have an SVT [supraventricular tachycardia] , and that means at times my resting heart rate of 55 goes to one 70. It's uncomfortable. It's not life-threatening. I was really concerned, oh, may I have underlying cardiomyopathy, valvular disease, coronary artery disease. So Paul Friedman and Peter Newsworthy said, Hey, we're going to take a six lead ECG wearable, send it to your home and just record a bunch of data and your activities of daily living. And then we buy 5G cell phone. We'll be collecting those six leads and we'll run it through all of our various validated AI systems. And then we'll tell you based on what the AI suggests, whether you're at high risk or not for various disease states. So it says your ejection fraction 70%. Oh, good. Don't have to worry about that. Your likelihood of developing AFib 3% cardiomyopathy, 2% valvular disease, 1%. So bottom line is without even going to a bricks and mortar facility here, I have these validated algorithms, at least doing a screen to see where maybe I should get additional evaluation and not.
Eric Topol (05:12):
Yeah, well see what you're bringing up is a whole other dimension. So on the one hand that what we talked about was you could give the primary care doctors who don't read electrocardiograms very well, you give them supercharged by having a deep learning interpretation set for them. But on the other, now you're bringing up this other patient facing story where you're taking a cardiogram when somebody's perfectly fine. But from that, from having deep learning of cardiograms, millions of cardiograms, you're telling what their risks are that they could develop things like atrial fibrillation. So this is starting to span the gamut of what the phase that we went through or still going through, which is taking medical images, whether it's a cardiogram or a scan of some sort, and seeing things with machines that humanize really can't detect or perceive. So yeah, we're just starting to get out of the block here, John. And you've already brought up a couple of major applications that we were not even potentially used three, four or five years ago that Mayo Clinics leading the charge, right?
The Power of Machine Eyes
John Halamka (06:26):
Well, yeah, and let me just give you two quick other examples of these are in studies now, right? So they're not ready for active patient use. The animate GI product does an overread of endoscopy. And what we're finding is that the expert human, I mean anywhere in the world, expert humans miss about 15% of small polyps. They're just hard to see. Prep may not be perfect, et cetera. The machine misses about 3%. So that's to say a human augmented with overread is five times better than a human alone pancreatic cancer, my father-in-law died about 11 years ago of stage four pancreatic cancer. So this is something that I'm very sensitive about, very often diagnosed late, and you can't do much. What we've been able to see is looking at pancreatic cancer, early films that were taken, abdominal CT scans and these sorts of things, algorithms can detect pancreatic cancer two years before it is manifested clinically. And so here's the ethical question I'll pose to you. I know you think about a lot of this Scripps Mayo, UCSF, Stanford, we probably have thousands and thousands of abdominal CTs that were read normal. Is it an ethical imperative as these things go through clinical trials and are validated and FDA approved to rerun algorithms on previous patients to diagnose disease we didn't see?
Eric Topol (08:03):
Well, that is a really big important question because basically we're relieving all this stuff on the table that doesn't get diagnosed, can't be predicted because we're not even looking for it. And now whether it's retina, that is a gateway to so many systems of the body, or as you're mentioning various scans like an abdominal CT and many others that like mammography for heart disease risk and all sorts of things that weren't even contemplated that machine eyes can do. So it's really pretty striking and upending cancer diagnosis, being able to understand the risk of any individual for particular types of cancer so that you can catch it at the earliest possible time when it's microscopic before it spreads. This, of course, is a cardinal objective. People don't die of cancer per se. They die of its metastasis, of course, for the most part. So that gets me now to the next phase of ai because what we've been talking for mostly so far has been what has been brewing culminating for the last five years, which is medical images and what, there's so many things we can glean from them that humans can't including expert humans in whatever discipline of medicine.
Multimodal AI and Social Determinants of Health
(09:19):
But the next phase, which you are starting to get at is the multimodal phase where you're not just taking the images, you're taking the medical records, the EHRs, you're getting the genomics, the gut microbiome, the sensors. You mentioned one, an ECGs, a cardiogram sensor, but other sensors like on the wrist, you're getting the environmental things like air pollution, air quality and various things. You're getting the whole ball of wax any given individual. Now, that's kind of where we're headed. Are you doing multimodal ai? Have you already embarked in that new path? Now that we have these large language models
John Halamka (10:02):
And we have, and so like anything we do in healthcare innovation, you need a Pareto diagram to say, what do you start with and where do you go? So in 2020, we started with all of the structured data problems, meds, allergies, labs. Then we went to the unstructured data, billions of notes, op reports, H and Ps, and then we moved to telemetry, and then we moved to CT, MRI, PET. Then we move to radiation oncology and looking at all the auto contouring profiles used in linear accelerators and then to omic, and now we're moving to an inferred social determinants of health. And let me explain that for a minute.
(10:45):
Exposome, as you point out, is really critical. Now, do you know if you live in a Superfund s
Melanie Mitchell: Straight Talk on A.I. Large Language Models
39m · PublishedTranscript with Links
Eric Topol (00:00):
This is Eric Topol, and I'm so excited to have the chance to speak to Melanie Mitchell. Melanie is the Davis Professor of Complexity at the Santa Fe Institute in New Mexico. And I look to her as one of the real, not just leaders, but one with balance and thoughtfulness in the high velocity AI world of large language models that we live in. And just by way of introduction, the way I got to first meet Professor Mitchell was through her book, Artificial Intelligence, A Guide for Thinking Humans. And it sure got me thinking back about four years ago. So welcome, Melanie.
Melanie Mitchell (00:41):
Thanks Eric. It's great to be here.
The Lead Up to ChatGPT via Transformer Models
Eric Topol (00:43):
Yeah. There's so much to talk about and you've been right in the middle of many of these things, so that's what makes it especially fun. I thought we'd start off a little bit of history, because when we both were writing books about AI back in 2019 publishing the world kind of changed since . And in November when ChatGPT got out there, it signaled there was this big thing called transformer model. And I don't think many people really know the difference between a transformer model, which had been around for a while, but maybe hadn't come to the surface versus what were just the deep neural networks that ushered in deep learning that you had so systematically addressed in your book.
Melanie Mitchell (01:29):
Right. Yeah. Transformers are, were kind of a new thing. I can't remember exactly when they came out, maybe 2018, something like that, right from Google. They were an architecture that showed that you didn't really need to have a recurrent neural network in order to deal with language. So that was one of the earlier things, you know, and Google translate and other language processing systems, people were using recurrent neural networks, networks that sort of had feedback from one time step to the next. But now we have the transformers, which instead use what they call an attention mechanism where the entire text that the system is dealing with is available all at once. And the name of the paper, in fact was Attention is All You need. And that by attention is all you need they meant this particular attention mechanism in the neural network, and that was really a revolution and enabled this new era of large language models.
Eric Topol (02:34):
Yeah. And as you aptly pointed out, that was in, that was five years ago. And then it took like, oh, five years for it to become in the public domain of Chat GPT. So what was going on in the background?
Melanie Mitchell (02:49):
Well, you know, the idea of language models (LLMs) that is neural network language models that learn by trying to predict the next word in a, in a text had been around for a long time. You know, we now have GPT-4, which is what's underlying at least some of ChatGPT, but there was GPT-1 and GPT-2, you probably remember that. And all of this was going on over those many years. And I think that those of us in the field have seen more of a progression with the increase in abilities of these increasingly large, large language models. that has really been an evolution. But I think the general public didn't have access to them and ChatGPT was the first one that like, was generally available, and that's why it sort of seemed to appear out of nothing.
SPARKS OF ARTIFICIAL GENERAL INTELLIGENCE
Sentience vs Intelligence
Eric Topol (03:50):
Alright. So it was kind of the, the inside world of the computer science kinda saw a more natural progression, but people were not knowing that LLMs were on the move. They were kinda stunned that, oh, look at these conversations I can have and how, how humanoid it seemed. Yeah. And you'll recall there was a fairly well-publicized event where a Google employee back I think last fall was, put on suspension, ultimately left Google because he felt that the AI was sentient. Maybe you'd want to comment that because that's kind of a precursor to some of the other things we're going to discuss,
Melanie Mitchell (04:35):
Right? So yeah, so one of the engineers who was working with their version of ChatGPT, which I think at the time was called LaMDA was having conversations with it and came to the conclusion that it was sentient, whatever that means, , you know, that, that it was aware that it had feelings that it experienced emotions and all of that. He was so worried about this and he wanted, you know, I think he made it public by releasing some transcripts of his conversations with it. And I don't think he was allowed to do that under his Google contract, and that was the issue. tThat made a lot of news and Google pushed back and said, no, no, of course it's not sentient. and then there was a lot of debate in the philosophy sphere of what sentient actually means, how you would know if something is sentient. And it Yeah. and it's kind of gone from there.
Eric Topol (05:43):
Yeah. And then what was interesting is then in March based upon GPT-4 the Microsoft Research Group published this sparks paper where they said, it seems like it has some artificial general intelligence, AGI qualities, kind of making the same claim to some extent. Right?
Melanie Mitchell (06:05):
Well, that's a good question. I mean, you know, intelligence is one thing, sentience is another. There's a question of whether, you know, how they're related, right? Or if they're related at all, you know, and what they all actually mean. And these terms, this is one of the problems. Of course, these terms are not well-defined, but most, I think most people in AI would say that intelligence and sentience are different. You know something can be intelligent or act intelligently without having any sort of awareness or sense of self or, you know, feelings or whatever sentience might mean. So I think that the sparks of AGI paper from Microsoft was more about this, that saying that they thought GPT-4 four, the system they were experimenting with, showed some kind of generality in its ability to deal with different kinds of tasks. You know, and this, this contrasts with the old, older fashioned ai, which typically was narrow only, could do one task, you know, could play chess, could play Go, could do speech recognition, or could, you know, generate translations. But it, they couldn't do all of those things. And now we have these language models, which seemed to have some degree of generality.
The Persistent Gap Between Humans and LLMs
Eric Topol (07:33):
Now that gets us perfectly to an important Nature feature last week which was called the “Easy Intelligence Test that AI chatbots fail.” And it made reference to an important study you did. First, I guess the term ARC --Abstract and Reasoning Corpus, I guess that was introduced a few years back by Francois Chollet. And then you did a ConceptARC test. So maybe you can tell us about this, because that seemed to have a pretty substantial gap between humans and GPT-4.
Melanie Mitchell (08:16):
Right? So, so, so Francois Chollet is a researcher at Google who put together this set of sort of intelligence test like puzzles visual reasoning puzzles that tested for abstraction abilities or analogy abilities. And he put it out there as a challenge. A whole bunch of people participated in a competition to get AI programs to solve the problems, and none of them were very successful. And so what, what our group did was we thought that, that the original challenge was fantastic, but the prob one of the problems was it was too hard, it was even hard for people. And also it didn't really systematically explore concepts, whether a, a system understood a particular concept. So, as an example, think about, you know, the concept of two things being the same, or two things being different. Okay?
(09:25):
So I can show you two things and say, are these the same or are they different? Well, it turns out that's actually a very subtle question. 'cause when we, you know, when we say the same we, we can mean sort of the, the same the same size, the same shape, the same color, this, you know, and there's all kinds of attributes in which things can be the same. And so what our system did was it took concepts like same versus different. And it tried to create lots of different challenges, puzzles that had that required understanding of that concept. So these are very basic spatial and semantic concepts that were similar to the ones that Solei had proposed, but much more systematic. 'cause you know, this is one of the big issues in evaluating AI systems is that people evaluate them on particular problems.
(10:24):
For example, you know, I think a lot of people know that ChatGPT was able to answer many questions from the bar exam. But if you take like a single question from the bar exam and think about what concept it's testing, it may be that ChatGPT could answer that particular question, but it can't answer variations that has the same concept. So we tried to take inside of this arc domain abstraction and reasoning corpus domain, look at particular concepts and say, systematically can the system understand different variations of the same concept? And then we tested this, these problems on humans. We tested them on the programs that were designed to solve the ARC challenges, and we tested them on G P T four, and we found that humans way outperformed all the machines. But there's a caveat, though, is that these are visual puzzles, and we're giving them to GPT-4, which is a language model, a text, right? Right. System. Now, GPT four has been trained on images, but we're not using the system that can deal with
Al Gore: The Intersection of A.I. and Climate Change
34m · PublishedTranscript with some hyperlinks
Eric Topol (00:00):
Hello, Eric Topol here. And what a privilege to have as my guest Al Gore, as we discuss things that are considered existential threats. And that includes not just climate change but also recently the concern about A.I. No one has done more on the planet to bring to the fore the concerns about climate change. And many people think that the 2006 film, An Inconvenient Truth, was the beginning, but it goes way back into the 1980s. So, Al it's really great to have you put in perspective. Here we are with the what's going on in Canada with more than 12 million acres of forest fires that are obviously affecting us greatly, no less the surface temperature of the oceans. And so many other signs of this climate change that you had warned us about decades ago are now accelerating. So maybe we could start off out, where are we with climate change and the climate reality?
The Good News on Climate Change
Al Gore (01:00):
Oh, well, first of all, thank you so much for inviting me to be on your podcast again, Eric. It's always a pleasure and especially because you're the host and we, we have very interesting conversations that aren't on the podcast. So, , I'm looking forward to this one. So, to start with climate you know, the old cliche, there's good news and bad news. Unfortunately, there's an abundance of bad news but there's also an awful lot of good news. Let me start with that first and then turn to the more worrying trends. We have seen the passage in the US last August of the largest and most effective best funded climate legislation passed by any nation in all of history. The so-called Inflation Reduction Act is an extraordinary piece of legislation.
(01:55):
It's billed as allocating $369 billion to climate solutions. But actually, the heavy lifting in that legislation is done by tax credits, most of which are open-ended and uncapped, and a few without any time limits, most a 10-year duration. And the enthusiastic response to the legislation after President Biden signed it has now made it clear that that early estimate of 369 billion is a low-ball estimate, because Goldman Sachs, for example, is predicting that it will end up allocating 1.2 trillion to climate solutions. A lot of other investors and others using economic models are estimating more than a trillion. So, it's really a fantastic piece of legislation and other nations are beginning to react and respond and copy it. One month after that law was passed the voters of Australia threw out their climate denying government and replaced it with a climate-friendly government, which immediately then set about passing legislation that adopts the same goals as the US IRA and the Australian context.
(03:19):
And they stopped the biggest new coal mine there. And anyway, one month after that, in October, the voters of Brazil threw out their former president often called the “Trump of the Tropics” and replaced him with a new president, a former president who's a new president, who has pledged to protect the Amazon and the European Union in responding to the evil, evil and cruel invasion of Ukraine by Russia. And the attempted blackmail of nations in Europe, dependent on Russian gas and oil responded not by bending their knee to Vladimir Putin, but by saying, wait a minute, this makes renewable energy, freedom, energy. And so they accelerated their transition. And so these are all excellent signs and qualifies as good news. The other good news is not all that new, but it's still continuing to improve.
(04:28):
And that is the astonishing reductions in cost for electricity produced by solar and wind, and the reductions in cost for energy storage, principally in batteries and electric vehicles and a hundred other less well known technologies that are extremely important. We're in the midst of early stages of a sustainability revolution that has the magnitude of the industrial revolution, coupled with the speed of the digital revolution. And we're seeing it all over the place. It’s really quite heartening. One quick example last, the, the biggest single source of global warming pollution is the generation of electricity with gas and coal. Well, last year, if you look at all the new electricity generation capacity installed worldwide 90% of it was renewable. In India, 93% was solar and wind. And India's pledged not to give permits for any new coal burning plants for at least five years, which means never, probably because this cost reduction curve, as I mentioned, is still continuing downward electric vehicles, we're now seeing that the purchases have reached 15% of the market globally.
(05:56):
Norway's already at 50%. They've actually outlawed the sale of any new internal combustion engines. And indeed, many national and even municipal and state jurisdictions have prospectively served notice that they, you won't be able to buy them after a certain day, 2030, in many cases and the auto companies and truck and bus companies have long since diverted their research money all their R & D is going into EVs now. And that's the second largest source of global warming pollution. I could go through the others, but I want, I'll just tell you that there is a lot of good news.
And the Bad News
Now, the bad news is we're still seeing the crisis get worse, faster than we're deploying all of these solutions. And, the inertia in our political and economic systems is partly a direct result of huge amounts of lobbying and campaign contributions and the century old net of political and economic influence built up by the fossil fuel industry.
(07:18):
And they're opposing every single solution at the state level, the local level, the national level, the international level. Now, this COP 28 [the 2023 United Nations Climate Change Conference] coming up at the end of the year in the United Arab Emirates is actually chaired by an oil and gas company CEO-- It's preposterous. And they already have in the last two COPS, more lobbyists registered as participants than all than the five or six largest national delegations combined. And we're seeing them really oppose this change. And meanwhile, the manifestations of the crisis are steadily worsening. You mentioned the fires in Canada that are predicted to burn all summer long. And I was in New York City last week, and you, you know, from the news stories it, it was horrific. I got there the day after the worst day, oh my God.
(08:21):
But I saw and heard from people just the tremendous problems that people have. It's also going on in Siberia, by the way, and these places that are typically beyond the reach of TV crews and networks that don't capture our attention unless something happens to blow the smoke to where we live. And that's what's happened here. But there are many other extremely worrying manifestations that aren't getting much attention. I do think we're going to solve this, Eric. I'm very optimistic, but the question is whether we will solve it in time. We are what's the right way to say this? We're tiptoeing through a minefield with tripwires and toward the edge of a cliff. I don't want to torture the metaphor, but actually there are several extremely dangerous threats to ecological systems that are in a state of balance now, and are being pushed out of their equilibrium state into a different format.
(09:35):
The ocean currents--we're already seeing it with the jet stream in the northern hemisphere. You may have seen on the weather maps. They're now using these a lot where it's getting loopier and more disorganized. That's what the last few winners has, has pulled these big loops, have pulled arctic air down into areas far south in the US and in other regions, by the way. And it’s making a lot of the extreme events worse. Now, we're entering an El Nino phase in the Pacific Ocean comes around every so often, and this one is predicted to be a strong one, and that's going to accentuate the temperature increase. You know, it was [recently] 110 degrees last week in Puerto Rico, 111 degrees in several countries in Southeast Asia.
(10:31):
Last summer, China had a heat wave that the historians say about, which the historians say there's nothing even minimally comparable in all prior known, and the length, the extent, the duration, the intensity. And we saw monsoons lead to much of Pakistan underwater for an extended period of time. I could go on, but the net and balance out the good news and the bad news we are gaining momentum. And soon we are going to be gaining on the crisis itself and start deploying solutions faster than it's getting worse. So I remain optimistic, and I always remind people, if you doubt we have the political will to see this through, remember that political will is itself a renewable resource.
The Intersection of A.I. and Climate Change
Eric Topol (11:27):
Yeah, that's a great optimistic point, and we sure appreciate that, because it's pretty scary to see these trends that you reviewed. Now, as you know recently there was a large group of AI scientists this one led by Sam Altman of OpenAI, who put out a statement, a one-sentence statement, and it said, “Mitigating the risk of distinction from ai, which you and are enthusiastic about, should be a global priority alongside other societal scale risks, such as pandemics and nuclear war.” Well, obviously, also climate change. So how do you see the AI intersection of climate change? Because as you well know, GPT-4, having pre-trained with some 30,000 graphic processing units [GPUs], the issues about consumption of energy carbon emissions, the need for water cooling, is AI going to make this situation worse, or will it make it better?
Al Gore (12:33):
Ground Truths has 33 episodes in total of non- explicit content. Total playtime is 23:41:12. The language of the podcast is English. This podcast has been added on February 4th 2024. It might contain more episodes than the ones shown here. It was last updated on May 29th, 2024 03:12.